High-speed train bearing fault diagnosis method based on ensemble learning
An integrated learning, high-speed train technology, applied in integrated learning, testing of mechanical components, testing of machine/structural components, etc., can solve problems such as low fault diagnosis accuracy, improve classification accuracy, improve denoising accuracy, high precision effect
- Summary
- Abstract
- Description
- Claims
- Application Information
AI Technical Summary
Problems solved by technology
Method used
Image
Examples
Embodiment
[0104] This embodiment is based on the integrated learning-based high-speed train bearing fault diagnosis method, which is specifically implemented according to the following steps:
[0105] The noisy original signal is fault marked and CEEMDAN decomposed, such as image 3 As shown, the CEEMDAN algorithm is an improved method for the modal aliasing phenomenon in the decomposition process of the EEMD algorithm, which can achieve better separation of the eigenmode functions, accurately reconstruct the original signal, and has a lower computational cost cost. After the signal is processed by CEEMDAN, the complex original signal will be decomposed into a series of eigenmode components IMF, each IMF component contains different frequency components, using the CEEMDAN algorithm to denoise the signal preprocessing can accurately separate the signal, The original signal is input into the CEEMDAN algorithm model, and after decomposition, 17 IMF components and 1 Res margin are obtained...
PUM
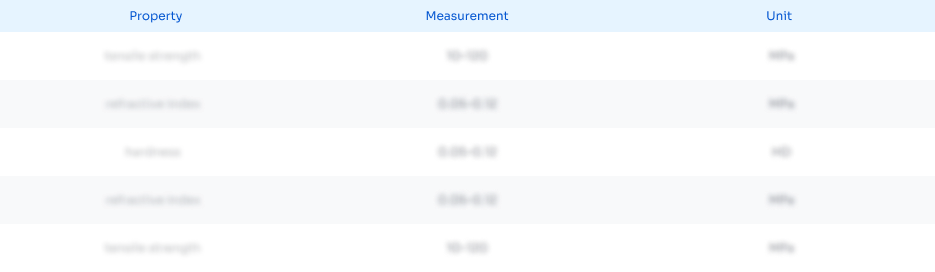
Abstract
Description
Claims
Application Information
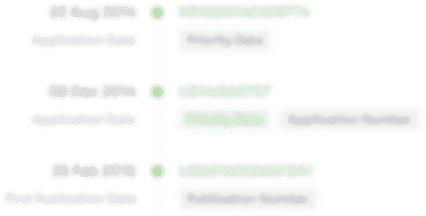
- R&D Engineer
- R&D Manager
- IP Professional
- Industry Leading Data Capabilities
- Powerful AI technology
- Patent DNA Extraction
Browse by: Latest US Patents, China's latest patents, Technical Efficacy Thesaurus, Application Domain, Technology Topic, Popular Technical Reports.
© 2024 PatSnap. All rights reserved.Legal|Privacy policy|Modern Slavery Act Transparency Statement|Sitemap|About US| Contact US: help@patsnap.com