Method for identifying discourse components based on graph neural network
A neural network and discourse technology, applied in the field of discourse component recognition based on graph neural network, can solve the problems of decreased text component recognition effect, increased training time, and reduced recognition effect of text component recognition system, and achieves faster speed. The speed of training, the effect of reducing the number of connected edges, and improving the effect
- Summary
- Abstract
- Description
- Claims
- Application Information
AI Technical Summary
Problems solved by technology
Method used
Image
Examples
Embodiment Construction
[0103] like figure 2 As shown, the present invention comprises the following steps:
[0104] Step 1: Build a text component recognition system. The system such as figure 1 As shown, it consists of a feature extraction module, a position encoding module, a discourse self-attention module, and a feature splicing module.
[0105] The feature extraction module is connected with the position encoding module and the discourse self-attention module, and its function is to extract sentence features. It is a deep feedforward neural network, which consists of a representation layer, a sequence encoding layer and a graph neural network layer. The feature extraction module summarizes the word feature information in the input article, obtains the feature representation of each sentence, uses the graph neural network to update the feature information of each sentence, obtains the feature representation of each sentence, and combines the features of each sentence. The representation is ...
PUM
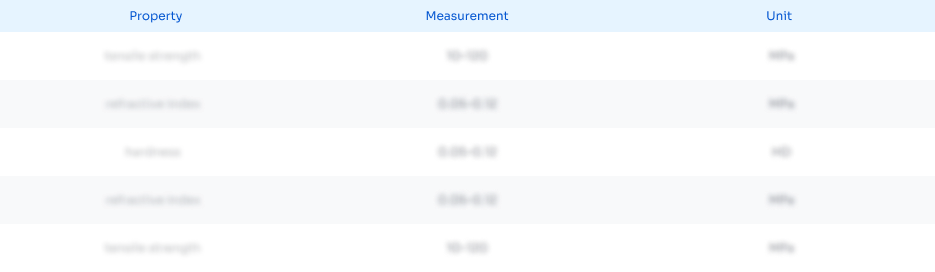
Abstract
Description
Claims
Application Information
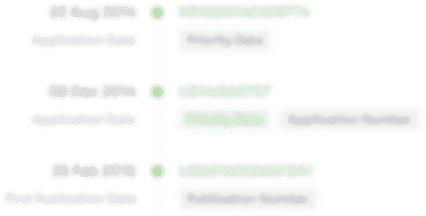
- R&D Engineer
- R&D Manager
- IP Professional
- Industry Leading Data Capabilities
- Powerful AI technology
- Patent DNA Extraction
Browse by: Latest US Patents, China's latest patents, Technical Efficacy Thesaurus, Application Domain, Technology Topic, Popular Technical Reports.
© 2024 PatSnap. All rights reserved.Legal|Privacy policy|Modern Slavery Act Transparency Statement|Sitemap|About US| Contact US: help@patsnap.com