Model set adaptation by probability mass diffusion
a probability mass diffusion and model technology, applied in the field of stochastic model prediction, can solve the problems of high computational burden, high possibility, complex computational task of prediction based on elliptical gaussian probability function, etc., and achieve the effect of easing computational burden
- Summary
- Abstract
- Description
- Claims
- Application Information
AI Technical Summary
Benefits of technology
Problems solved by technology
Method used
Image
Examples
Embodiment Construction
[0112]The invention concerns a new concept—denoted the “probability mass diffusion” (PMD) principle—for re-arranging the model set after the measurement updates.
[0113]The present invention, shall initially be explained in more detail by focusing on the computational workings and effects in close relation to the IMM method as applied to a number of exemplary radar applications, while later expanding the description to general applications where recursive estimation of dynamic systems / signals are used.
[0114]For this purpose we shall initially focus on four examples, in which the same complementary model / subset model is used for the PMD method and the IMM method, respectively and where the measurements fall either clearly within a given model or in an ambiguous area between models, respectively.
[0115]The indices are the same as defined above for FIGS. 16-21, except that being expanded with suffix a denoting the re-arranged model sets.
[0116]The IMM and the proposed method are equal in t...
PUM
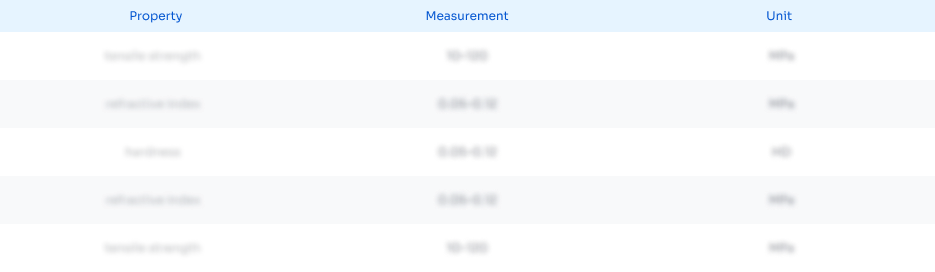
Abstract
Description
Claims
Application Information
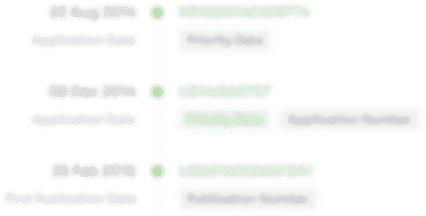
- R&D
- Intellectual Property
- Life Sciences
- Materials
- Tech Scout
- Unparalleled Data Quality
- Higher Quality Content
- 60% Fewer Hallucinations
Browse by: Latest US Patents, China's latest patents, Technical Efficacy Thesaurus, Application Domain, Technology Topic, Popular Technical Reports.
© 2025 PatSnap. All rights reserved.Legal|Privacy policy|Modern Slavery Act Transparency Statement|Sitemap|About US| Contact US: help@patsnap.com