Hypothesis-driven classification of materials using nuclear magnetic resonance relaxometry
a nuclear magnetic resonance and relaxometry technology, applied in the direction of nuclear magnetic resonance analysis, measurement using nmr, instruments, etc., can solve the problems of difficult to distinguish hazardous materials from non-hazardous materials, different hazardous materials from each other, and measurement has a cost in required energy, result processing, time and other resources, and achieves low overall measurement cost, high degree of confidence, and the effect of costing the required energy
- Summary
- Abstract
- Description
- Claims
- Application Information
AI Technical Summary
Benefits of technology
Problems solved by technology
Method used
Image
Examples
example 1
[0030]An exemplary method 300 for determining the identity of a substance is illustrated in FIG. 3. Method 300 may be implemented at least in part by a computing device. A reference group of known materials is identified in step 302, the known materials having known values for classification parameters. The classification parameters comprise at least one of T1, T2, T1ρ, RNS, and LAC. In step 304, a measurement sequence is optimized based on at least one of a measurement cost of the classification parameters and an initial probability of the known materials in the reference group.
[0031]In some examples at least one classification parameter value is obtained for the substance according to the optimized measurement sequence. The at least one classification parameter value obtained for the substance may then be compared to classification parameter values of the known materials in the reference group to determine whether the substance is one of the known materials. In some examples, comp...
example 2
[0035]An exemplary method 400 for determining the identity of a substance is illustrated in FIG. 4. Method 400 may be implemented at least in part by a computing device. In step 402, a set of classification parameters for which values can be obtained is determined. The classification parameters comprise at least one of T1, T2, T1ρ, RNS, and LAC. In step 404, a measurement cost of each classification parameter in the set is determined. Measurement cost may relate to the time required to perform the measurement and obtain classification parameter values.
[0036]A reference group of known materials each having known classification parameter values is identified in step 406. In step 408, an initial probability is received for each material in the reference group. A plurality of measurement sequences for obtaining values for each of the set of classification parameters for the substance is determined in step 410. An aggregate cost for each of the plurality of measurement sequences is calcu...
example 3
[0039]An exemplary method 500 for building one or more substance identification tables for determining the identity of a substance are illustrated in FIG. 5. Method 500 may be implemented at least in part by a computing device. In step 502, a set of classification parameters for which values can be obtained is determined. The classification parameters comprise at least one of T1, T2, T1ρ, RNS, and LAC. In step 504, a measurement cost of each classification parameter in the set is determined. The measurement cost reflects the time required to obtain a measurement value of the classification parameter. In step 506, a reference group of known materials is identified. Classification parameter values for each of the known materials in the reference group are obtained in step 508. An initial probability is assigned to each material in the reference group in step 510. Such a substance identification table can be used to identify substances as discussed above with regard to methods 100, 200...
PUM
Property | Measurement | Unit |
---|---|---|
NMR | aaaaa | aaaaa |
linear attenuation coefficient | aaaaa | aaaaa |
attenuation coefficient | aaaaa | aaaaa |
Abstract
Description
Claims
Application Information
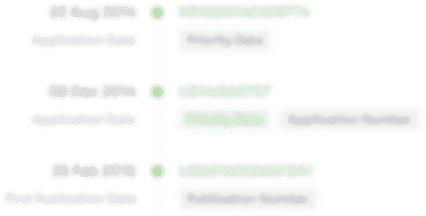
- R&D Engineer
- R&D Manager
- IP Professional
- Industry Leading Data Capabilities
- Powerful AI technology
- Patent DNA Extraction
Browse by: Latest US Patents, China's latest patents, Technical Efficacy Thesaurus, Application Domain, Technology Topic, Popular Technical Reports.
© 2024 PatSnap. All rights reserved.Legal|Privacy policy|Modern Slavery Act Transparency Statement|Sitemap|About US| Contact US: help@patsnap.com