An increment type characteristic background modeling algorithm of self-adapting weight selection
A background modeling and self-adaptive technology, applied in computing, computer components, character and pattern recognition, etc., can solve problems such as interpretation, algorithm accuracy is greatly affected, and weight calculation methods are not provided
- Summary
- Abstract
- Description
- Claims
- Application Information
AI Technical Summary
Problems solved by technology
Method used
Image
Examples
Embodiment 1
[0055] Example of background modeling for speedboats on the West Lake:
[0056] The first scene is the West Lake reflected in the setting sun, and a speedboat speeds across the lake in the distance. There are certain difficulties in motion detection in this scene: First, the foreground object we want to detect, that is, the speedboat, occupies too small an area in the entire picture, and the movement of the speedboat is easily absorbed by the background model as background noise; Secondly, the background changes in the video screen are more significant, including the leaves blown by the wind near the camera and the sunlight reflected by the lake waves. as shown in picture 2.
[0057] The motion detection results are shown in Figure 3. (a) and (b) are the background image and the detected foreground area produced by the traditional feature background modeling method, (c) and (d) are the background image and the foreground area obtained by the method of the present invention. ...
Embodiment 2
[0059] Example of background modeling for the moving human body by the West Lake:
[0060] The second scene is a person walking along the Su Causeway by the lake, and the background is the West Lake with wind and wave movement, as shown in Figure 4(a). The difficulty here is that the background lake has a large area, and the background wave motion is very complicated. At the same time, the nearby willow branches are dancing with the wind, which can easily be regarded as foreground objects. Based on this scene, we tested the traditional feature background modeling method and the method of the present invention respectively, and the generated background images are shown in Fig. 4(b) and Fig. 4(c) respectively. Figure 4(b) shows that there are obvious "ghosting" phenomena in the area where people pass by in the background image. This is because the traditional method treats the video frame as a whole without considering the motion of different areas in the image. Therefore, the ...
Embodiment 3
[0062] Example of background modeling for large-scale complex scenes:
[0063]The last scenario is challenging for all background modeling based motion detection methods. The test video was shot on the lawn of the Yuquan Campus of Zhejiang University. Someone ran quickly across the entire scene. The scene contained very complex background changes, such as irregularly moving crowds in the distance and bushes swaying in the wind. The traditional method and the method of the present invention are also tested based on this scenario, and Fig. 5 shows the effect of the algorithm. In Fig. 5, the first row is the 1373rd, 1410th, 1450th, 1634th and 1660th frame of the original video, the second row is the corresponding background image generated by the traditional method, and the third row is generated by the method of the present invention Corresponds to the background image. In the second row of images, the image area in the circle has a very obvious "ghosting" effect, which is als...
PUM
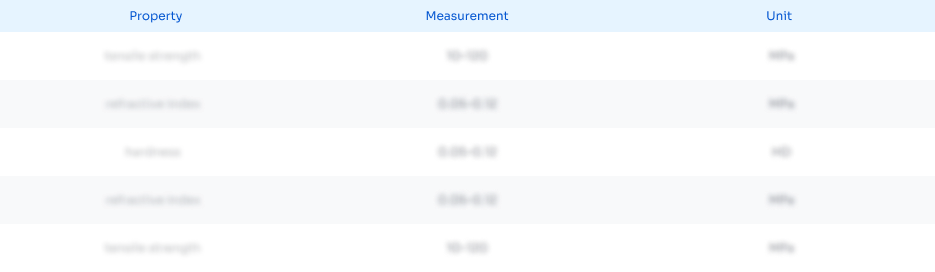
Abstract
Description
Claims
Application Information
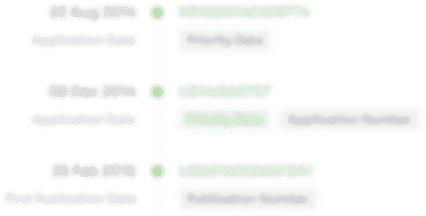
- R&D Engineer
- R&D Manager
- IP Professional
- Industry Leading Data Capabilities
- Powerful AI technology
- Patent DNA Extraction
Browse by: Latest US Patents, China's latest patents, Technical Efficacy Thesaurus, Application Domain, Technology Topic, Popular Technical Reports.
© 2024 PatSnap. All rights reserved.Legal|Privacy policy|Modern Slavery Act Transparency Statement|Sitemap|About US| Contact US: help@patsnap.com