Extensible self-adapting multi-core classification method
A classification method and self-adaptive technology, which is applied in the directions of instrumentation, computing, character and pattern recognition, etc., can solve problems such as complex feature representation, achieve robust classification effects, improve classification accuracy, and solve data aliasing problems
- Summary
- Abstract
- Description
- Claims
- Application Information
AI Technical Summary
Problems solved by technology
Method used
Image
Examples
Embodiment Construction
[0039] The present invention will be further described below in conjunction with the accompanying drawings and specific embodiments.
[0040] figure 2 is a workflow diagram according to an embodiment of the present invention. Using the present invention to solve complex image classification problems, taking the Caltech256 image data set as an example, the data set contains 257 categories of image data, wherein each category of images contains more than 80 image samples. During the implementation, 30 samples of each class are selected for training and correction, and the remaining samples are used for testing. After all the image samples are extracted from features such as color, texture, and shape, the steps of utilizing the present invention to realize image classification are as follows (work flow chart is shown in figure 2 ):
[0041] Step 1, preprocessing stage
[0042] Using the PLSA (probabilistic latent semantic analysis) method to perform heuristic unsupervised c...
PUM
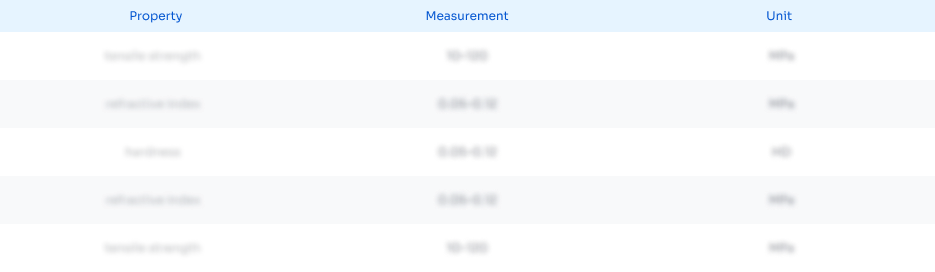
Abstract
Description
Claims
Application Information
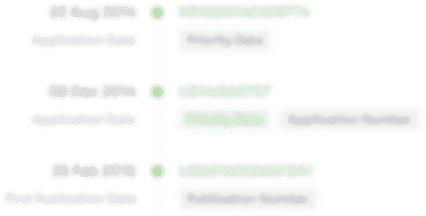
- R&D Engineer
- R&D Manager
- IP Professional
- Industry Leading Data Capabilities
- Powerful AI technology
- Patent DNA Extraction
Browse by: Latest US Patents, China's latest patents, Technical Efficacy Thesaurus, Application Domain, Technology Topic, Popular Technical Reports.
© 2024 PatSnap. All rights reserved.Legal|Privacy policy|Modern Slavery Act Transparency Statement|Sitemap|About US| Contact US: help@patsnap.com