A Method for Automatic Image Annotation and Translation Based on Decision Tree Learning
An automatic image and decision tree technology, applied in special data processing applications, instruments, electrical digital data processing, etc., can solve problems such as inadaptable image databases, incomplete databases, noisy data, etc.
- Summary
- Abstract
- Description
- Claims
- Application Information
AI Technical Summary
Problems solved by technology
Method used
Image
Examples
Embodiment
[0035] Given 5100 Corel database images, 570 images of 19×30 are selected as the training image set of the method of the present invention, and the embodiment performs automatic image labeling on the remaining images.
[0036] (1) Segment all images in the training image set to form several image sub-blocks (regions), extract color, texture, and shape features from the image sub-blocks, and obtain feature data x 1 , x 2 ,...,x L (L-dimensional color feature), y 1 ,y 2 ,...,y M (M-dimensional texture features), z 1 ,z 2 ,...,z N (N-dimensional shape features).
[0037] In the stage of discretization of eigenvalues processed by adaptive VQ, taking color features as an example, the first step is to calculate the initial clustering center, let this center be c 1 , and then set the initial number of clusters CN=1; the second step first selects the cluster centers that exceed the L-dimensional color feature, let n be the number of selected centers, if n=0, stop, otherwise ...
PUM
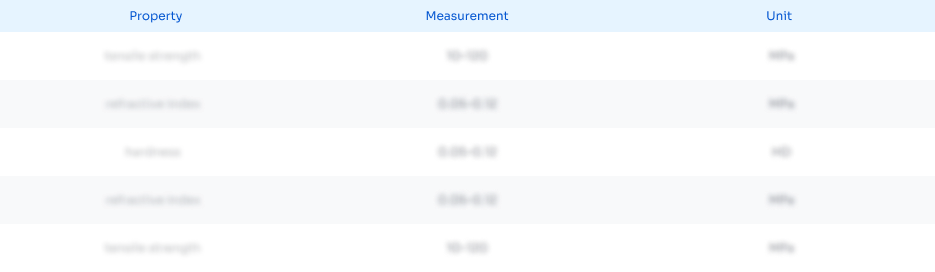
Abstract
Description
Claims
Application Information
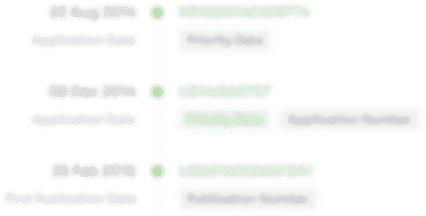
- R&D
- Intellectual Property
- Life Sciences
- Materials
- Tech Scout
- Unparalleled Data Quality
- Higher Quality Content
- 60% Fewer Hallucinations
Browse by: Latest US Patents, China's latest patents, Technical Efficacy Thesaurus, Application Domain, Technology Topic, Popular Technical Reports.
© 2025 PatSnap. All rights reserved.Legal|Privacy policy|Modern Slavery Act Transparency Statement|Sitemap|About US| Contact US: help@patsnap.com