Method and system for predicting flow of self-adaptive differential auto-regression moving average model
A technology of moving average and flow forecasting, applied in transmission systems, digital transmission systems, electrical components, etc., can solve the problems that the model is no longer applicable and cannot predict the flow value, etc., and achieve the effect of improving accuracy
- Summary
- Abstract
- Description
- Claims
- Application Information
AI Technical Summary
Problems solved by technology
Method used
Image
Examples
Embodiment Construction
[0034] The present invention will be further described below in conjunction with the accompanying drawings and embodiments.
[0035] Embodiment of Traffic Forecasting Method Based on Adaptive Differential Autoregressive Moving Average Model
[0036] figure 2 The flowchart of an embodiment of the traffic forecasting method of the adaptive differential autoregressive moving average model of the present invention is shown. See figure 2 , the following is a detailed description of each step in the method of this embodiment.
[0037] Step S100: Obtain an initial differential autoregressive moving average ARIMA model through learning from historical data.
[0038] ARIMA is a typical time series analysis algorithm, which brings historical data into the model for learning and determines the parameters of the model. The purpose of learning historical data is to determine the parameters of the model so that the subsequent real-time data can be directly brought into the ARIMA wit...
PUM
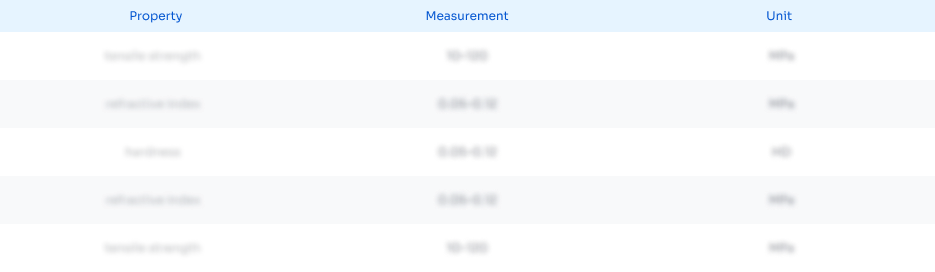
Abstract
Description
Claims
Application Information
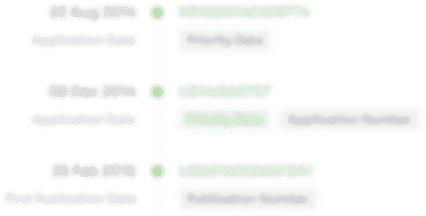
- R&D Engineer
- R&D Manager
- IP Professional
- Industry Leading Data Capabilities
- Powerful AI technology
- Patent DNA Extraction
Browse by: Latest US Patents, China's latest patents, Technical Efficacy Thesaurus, Application Domain, Technology Topic.
© 2024 PatSnap. All rights reserved.Legal|Privacy policy|Modern Slavery Act Transparency Statement|Sitemap