Interval forecasting method of heat supply load based on support vector machine and error estimation
A support vector machine, load interval technology, applied in computing, computer parts, character and pattern recognition, etc., can solve problems such as inability to apply heating energy-saving renovation, thermal scheduling and thermal station control, and low forecast accuracy
- Summary
- Abstract
- Description
- Claims
- Application Information
AI Technical Summary
Problems solved by technology
Method used
Image
Examples
specific Embodiment approach 1
[0064] Specific implementation mode one: as figure 1 As shown, the heating load interval forecasting method based on support vector machine and error estimation described in this embodiment is implemented according to the following steps:
[0065] Step 1. Establish a support vector regression forecasting model on the basis of obtaining sample data, and perform point forecasting:
[0066] Step 1 (1), sample data and training sample construction:
[0067] Suppose the heating load time series {L t (i)}, wherein, i=1,2,...,24, t=1,2,...,n 2 , here, n 2 is the number of days of the heating load collected;
[0068] For the current heating load L t (i), which can be obtained from the previous m of its current heating load 1 load value forecast, that is, L t (i-m 1 ), L t (i-(m 1 -1)), ..., L t (i-1)(i>m 1 );or
[0069] {L t (1), L t (2),...,L t (i-1); L t-1 [24-(m 1 -(i-1))],...,L t-1 (23), L t-1 (24)}, (1≤i≤m 1 )
[0070] The first m of the current heating load...
specific Embodiment approach
[0121] During the heating period from the winter of 2007 to the spring of 2008, a certain heat source was sampled every 1 hour, and the data obtained from the average daily outdoor temperature during the same period were used as the original data for the load forecast of the heating system, such as figure 2 and image 3 As shown, the specific process is:
[0122] 1. Sample data selection and sample input dimension determination
[0123] Using the pseudo-neighborhood method to calculate the input dimension of the horizontal and vertical forecasts, m 1 = 4, m 2 = 3, form the training sample recursive formula, and obtain the training sample {x i ,y i}.
[0124]
[0125] (15)
[0126]
[0127] 2. Support vector regression point forecast parameter selection
[0128] The accuracy of support vector regression prediction depends on the selection of model parameters. In this paper, the SVR kernel function chooses the radial basis function, that is, K(x j , x l )=exp(-||...
PUM
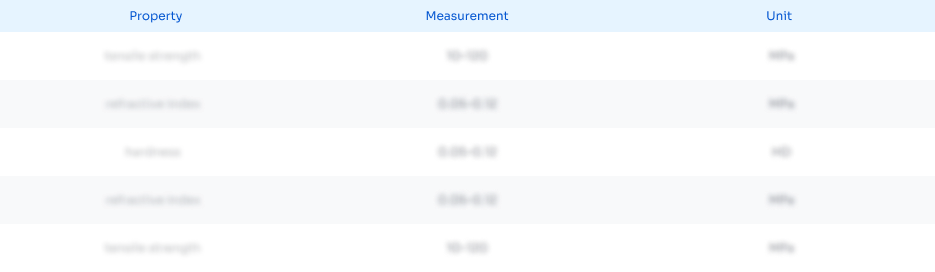
Abstract
Description
Claims
Application Information
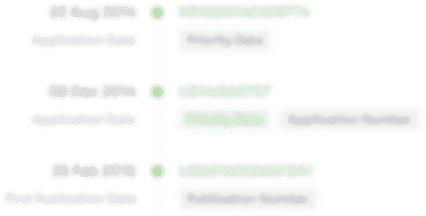
- R&D Engineer
- R&D Manager
- IP Professional
- Industry Leading Data Capabilities
- Powerful AI technology
- Patent DNA Extraction
Browse by: Latest US Patents, China's latest patents, Technical Efficacy Thesaurus, Application Domain, Technology Topic, Popular Technical Reports.
© 2024 PatSnap. All rights reserved.Legal|Privacy policy|Modern Slavery Act Transparency Statement|Sitemap|About US| Contact US: help@patsnap.com