Neural network sample selection method and device based on active learning
A neural network and active learning technology, applied in the field of machine learning, can solve problems such as high algorithm complexity and inapplicability, achieve the effect of reducing the number of training samples, reducing time and cost, and improving classification effects
- Summary
- Abstract
- Description
- Claims
- Application Information
AI Technical Summary
Problems solved by technology
Method used
Image
Examples
Embodiment Construction
[0024] The present invention will be described in detail below in conjunction with the accompanying drawings and specific embodiments.
[0025] Taking the multi-layer perceptron neural network as an example, the method for selecting samples of the feedforward neural network according to the present invention is described. However, those skilled in the art should understand that the present invention is not limited to MLP neural networks, but can be applied to other feed-forward neural networks.
[0026] MLP is a fully connected feed-forward neural network suitable for object classification. The structure of the MLP is as figure 1 As shown, it is a three-layer feed-forward network: the input layer MA is composed of input pattern nodes, x i Represents the i-th component of the input pattern vector (i=1, 2, ..., n); the second layer is the hidden layer MB, which consists of m nodes b j (j=1, 2, . . . , m) composition. The third layer is the output layer MC, which consists of ...
PUM
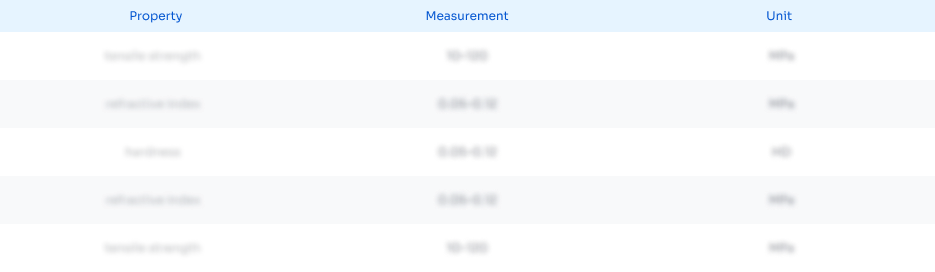
Abstract
Description
Claims
Application Information
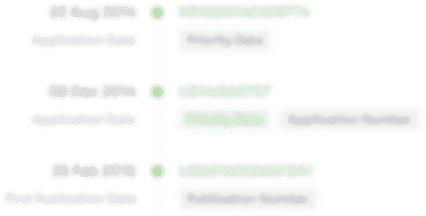
- R&D Engineer
- R&D Manager
- IP Professional
- Industry Leading Data Capabilities
- Powerful AI technology
- Patent DNA Extraction
Browse by: Latest US Patents, China's latest patents, Technical Efficacy Thesaurus, Application Domain, Technology Topic, Popular Technical Reports.
© 2024 PatSnap. All rights reserved.Legal|Privacy policy|Modern Slavery Act Transparency Statement|Sitemap|About US| Contact US: help@patsnap.com