Radial basis function (RBF) neural network parameter self-optimizing-based multi-step prediction method for water quality
A neural network, multi-step prediction technology, applied in biological neural network models, predictions, data processing applications, etc., can solve the problem that the water quality early warning system cannot provide sufficient emergency processing time, and achieve efficient and intelligent automatic early warning and improvement effects. Effect
- Summary
- Abstract
- Description
- Claims
- Application Information
AI Technical Summary
Problems solved by technology
Method used
Image
Examples
Embodiment
[0047] Such as figure 1 As shown, the water quality parameters (take pH as an example) of online monitoring of water pipe network in a certain place from November 10 to November 19, 2011 are taken. The real-time monitoring equipment monitors once every 15 minutes. There are 960 data in total. 768 data are used as the prediction training set, and 192 data of the last 2 days are used as the test set. Let the time series be , in order to ensure that the built neural network has sufficient input sensitivity and good fit for the samples, according to Normalize the training set, where and are the maximum and minimum values of the training set, respectively. Then calculate the autocorrelation coefficient of the pH training set sequence , set the threshold of the correlation coefficient is 0.9, such as figure 2 As shown, when time autocorrelation coefficient , so the input variables of the RBF neural network can be constructed as , the input-output pair of the tr...
PUM
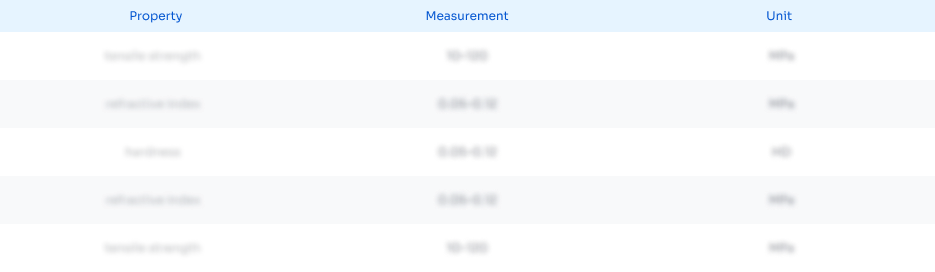
Abstract
Description
Claims
Application Information
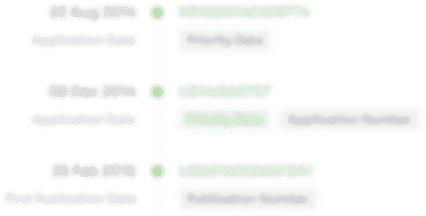
- R&D Engineer
- R&D Manager
- IP Professional
- Industry Leading Data Capabilities
- Powerful AI technology
- Patent DNA Extraction
Browse by: Latest US Patents, China's latest patents, Technical Efficacy Thesaurus, Application Domain, Technology Topic, Popular Technical Reports.
© 2024 PatSnap. All rights reserved.Legal|Privacy policy|Modern Slavery Act Transparency Statement|Sitemap|About US| Contact US: help@patsnap.com