Lithium ion battery residual life forecasting method of dynamic gray related vector machine
A correlation vector machine, lithium-ion battery technology, applied in the measurement of electricity, measurement of electrical variables, measurement devices, etc., can solve problems such as poor prediction accuracy, and achieve the effect of improving accuracy, strong generalization ability, and fixing hyperparameters
- Summary
- Abstract
- Description
- Claims
- Application Information
AI Technical Summary
Problems solved by technology
Method used
Image
Examples
specific Embodiment approach 1
[0036] Specific implementation mode 1. Combination figure 1 Specifically illustrate the present embodiment, the lithium-ion battery remaining life prediction method of the dynamic gray correlation vector machine described in the present embodiment, comprises the following steps:
[0037] Step 1, select the lithium-ion battery capacity training data, and use this data set as the original data set;
[0038] Step 2, MDGM modeling, predicting the original data set;
[0039] Step 3. Update the elements in the original data set according to the prediction results;
[0040] Step 4. Determine whether the original data prediction is completed, if so, perform step 5; if not, repeat the prediction;
[0041] Step 5, according to the input is the predicted value set of the original data, the output is the original data set, and train the correlation vector machine model;
[0042] Step 6. Capacity prediction: perform short-term prediction based on the MDGM model obtained in step 2, and ...
specific Embodiment approach 2
[0060] Embodiment 2. The difference between this embodiment and the lithium-ion battery remaining life prediction method of the dynamic gray correlation vector machine described in Embodiment 1 is that the lithium-ion battery capacity training data is selected in step 1, and the data is The specific process of the collection as the original data collection is:
[0061] Select the lithium-ion battery capacity data set X of 60 charge-discharge cycles before the current moment (0) ={x (0) (1),x (0) (2),...x (0) (i)...,x (0) (n)} as the original data set,
[0062] Among them, n=60.
specific Embodiment approach 3
[0063] Specific embodiment three. The difference between this embodiment and the lithium-ion battery remaining life prediction method of the dynamic gray correlation vector machine described in specific embodiment two is that the MDGM modeling described in step two, the specific method of predicting the original data set The process is:
[0064] A. Establish the gray differential equation: use the selected original data set as the input data set of the MDGM(1,1) model, and obtain x according to the formula (1) (1) (k):
[0065] x ( 1 ) ( k ) = Σ i = 1 k x ( 0 ) ( i ) , - - - ...
PUM
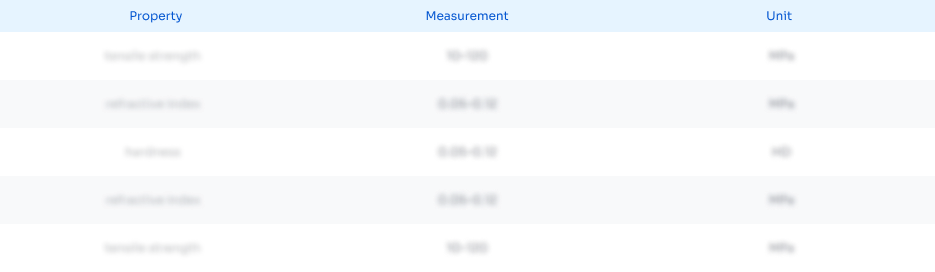
Abstract
Description
Claims
Application Information
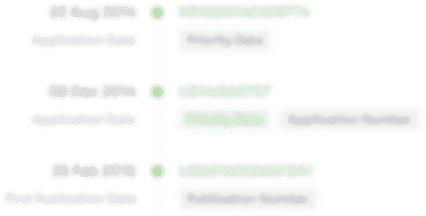
- Generate Ideas
- Intellectual Property
- Life Sciences
- Materials
- Tech Scout
- Unparalleled Data Quality
- Higher Quality Content
- 60% Fewer Hallucinations
Browse by: Latest US Patents, China's latest patents, Technical Efficacy Thesaurus, Application Domain, Technology Topic, Popular Technical Reports.
© 2025 PatSnap. All rights reserved.Legal|Privacy policy|Modern Slavery Act Transparency Statement|Sitemap|About US| Contact US: help@patsnap.com