A data dimension reduction method based on a tensor global-local preserving projection
A technology of locally preserving projection and data dimensionality reduction, applied in the fields of pattern recognition and machine learning, it can solve problems such as poor dimensionality reduction effect and only focusing on the global structure or local structure of the data.
- Summary
- Abstract
- Description
- Claims
- Application Information
AI Technical Summary
Problems solved by technology
Method used
Image
Examples
example
[0068] Example: Take face recognition as an example to implement, and use the classic ORL face database to verify the performance of the method proposed in the present invention. The ORL face dataset consists of 400 face images, including 40 people, 10 images per person, which were taken at different times, different lighting, different expressions and different facial details. The size of each image is 112 pixels by 92 pixels. Select 10 of them in the ORL library, randomly select 2 images from each person as training samples to form the training sample set X, and randomly select the other 5 images as test samples to form the test sample set T. Then the training set X={X 1 ,X 2 ,...,X i ,...,X 20},in test set T = {T 1 , T 2 ,...,T i ,...,T 50},in
[0069] First, use the method of the present invention to reduce the training set X to l×l dimension (l1 ,Y 2 ,...,Y i ,...,Y 20} Among them, in step (3), the k-nearest neighbor method is used to define the neighbor...
PUM
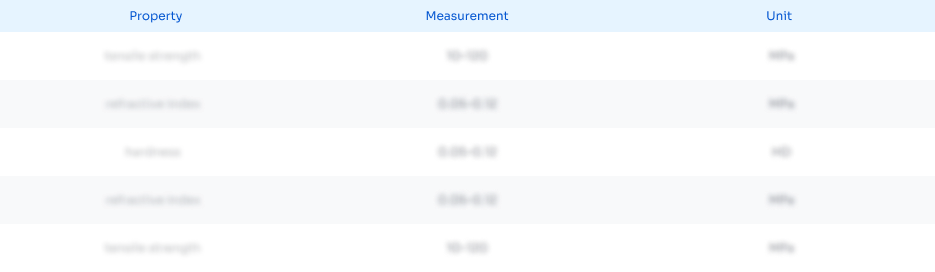
Abstract
Description
Claims
Application Information
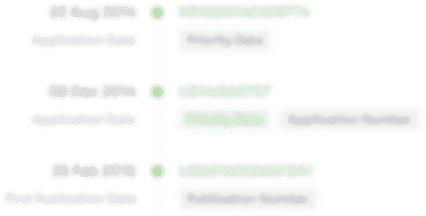
- R&D
- Intellectual Property
- Life Sciences
- Materials
- Tech Scout
- Unparalleled Data Quality
- Higher Quality Content
- 60% Fewer Hallucinations
Browse by: Latest US Patents, China's latest patents, Technical Efficacy Thesaurus, Application Domain, Technology Topic, Popular Technical Reports.
© 2025 PatSnap. All rights reserved.Legal|Privacy policy|Modern Slavery Act Transparency Statement|Sitemap|About US| Contact US: help@patsnap.com