Data training method of deep stack type hybrid self-encoding network
A self-encoding network and data training technology, applied in the field of small-sample photovoltaic hotspot recognition, can solve the problems of unbalanced sample set, inability to directly use classification neural network, insufficient original samples, etc., to achieve powerful feature extraction and expression capabilities, and enhance Robustness and generalization ability, overcoming the effect of insufficient sample size
- Summary
- Abstract
- Description
- Claims
- Application Information
AI Technical Summary
Problems solved by technology
Method used
Image
Examples
Embodiment
[0042] Example: such as figure 1 Shown, the data training method of a kind of depth stack type hybrid self-encoder network of the present invention comprises the following several steps;
[0043] Step 1. Perform image preprocessing on the collected photovoltaic infrared images to obtain a small sample hot spot image dataset;
[0044] Step 2. Use the small-sample hot spot image data set as a training sample, use the training sample to train DAE, learn the low-dimensional features of the image through encoding, perform input reconstruction through decoding and use the backpropagation algorithm to minimize the reconstruction error, When the reconstruction error reaches the minimum, it means that the DAE training is completed, and the structure and weight of the coding part at this time are retained;
[0045]Step 3, using the image low-dimensional features obtained in the DAE encoding process as the input of SAE, training SAE with the same method, and obtaining image sparse featu...
PUM
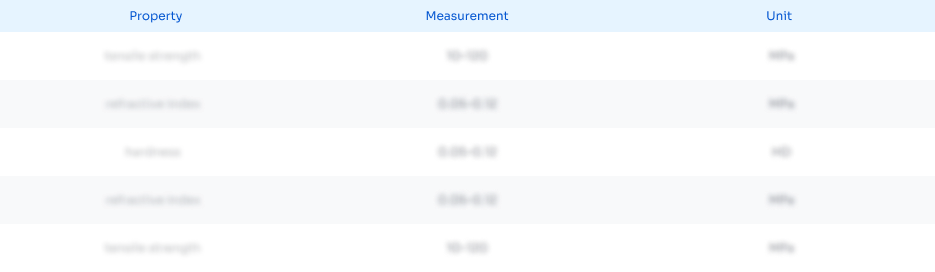
Abstract
Description
Claims
Application Information
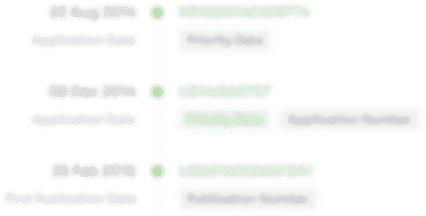
- R&D Engineer
- R&D Manager
- IP Professional
- Industry Leading Data Capabilities
- Powerful AI technology
- Patent DNA Extraction
Browse by: Latest US Patents, China's latest patents, Technical Efficacy Thesaurus, Application Domain, Technology Topic, Popular Technical Reports.
© 2024 PatSnap. All rights reserved.Legal|Privacy policy|Modern Slavery Act Transparency Statement|Sitemap|About US| Contact US: help@patsnap.com