Anomaly detection method based on multi-dimensional Epanechnikov kernel density estimation
A technology of kernel density estimation and anomaly detection, applied in electrical components, wireless communication, network topology, etc., can solve problems such as high computational complexity and failure to meet accuracy requirements, achieve broad application prospects, reduce communication overhead, and improve accuracy Effect
- Summary
- Abstract
- Description
- Claims
- Application Information
AI Technical Summary
Problems solved by technology
Method used
Image
Examples
Embodiment 1
[0075] The distributed data flow model involved in this implementation is as follows: figure 1 shown, from figure 1 It can be seen that there are a total of l+1 distribution nodes (including a cluster head node) in the sensor network. Each distribution node in the area will collect data at a certain period to form a data stream. In order to ensure the correctness of data samples collected by distributed nodes in this area, each distributed node needs to eliminate outliers in the sliding window before uploading data.
[0076] Now take the temperature value (unit is Celsius) as an example, assuming that 10 distribution nodes (V 1 to V 10 ), the sliding window width of each distribution node is N=20, so that the data collected by 10 distribution nodes within 20 moments constitutes a 10×20 sample matrix Z:
[0077] Z=(10.18, 10.56, 11.33, 19.56, 20.6, 9.05, 10.36, 10.79, 11.59, 9.44, 10.6, 10.07, 10.73, 9.35, 10.02, 11.4, 8.2, 11.78, 11.1, 10.57;
[0078] 10.86, 9.1, 9.86, 9....
PUM
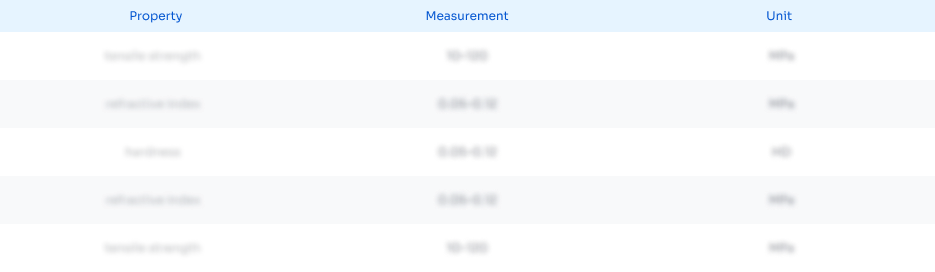
Abstract
Description
Claims
Application Information
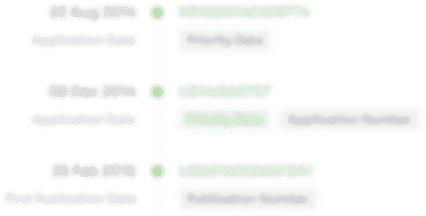
- R&D Engineer
- R&D Manager
- IP Professional
- Industry Leading Data Capabilities
- Powerful AI technology
- Patent DNA Extraction
Browse by: Latest US Patents, China's latest patents, Technical Efficacy Thesaurus, Application Domain, Technology Topic.
© 2024 PatSnap. All rights reserved.Legal|Privacy policy|Modern Slavery Act Transparency Statement|Sitemap