Method for face recognition by adopting two-dimensional non-negative sparse partial least squares
A partial least squares, non-negative sparse technology, applied in the field of two-dimensional non-negative sparse partial least squares for face recognition, it can solve the problems that the algorithm does not consider the non-negative constraints and the results are not interpretable enough.
- Summary
- Abstract
- Description
- Claims
- Application Information
AI Technical Summary
Problems solved by technology
Method used
Image
Examples
Embodiment 1
[0095] Example 1: for figure 1 (a) is performed on 11 original images of a person without occlusion, and the highest recognition rate results are as follows figure 2shown. It can be seen from this that the method of 2DNSPLS [the method of the present invention, that is, when the face training sample set X is projected on the base matrix, the non-negativity constraint and the sparsity constraint are considered] and the method of 2DNPLS [two-dimensional non-negative partial least squares Multiplication (Two-dimensional Nonnegative PLS, 2DNPLS, that is, when the face training sample set X is projected on the base matrix, only the non-negativity constraint is considered, and the converged base matrix is finally obtained through the iterative format (5) iteration] when there are fewer training samples The results are better than other two-dimensional algorithms. The 2DLDA and 2DLPP algorithms perform poorly when the training samples are small. In general, 2DNSPLS and 2DNPLS are...
Embodiment 2
[0096] Embodiment 2: This embodiment is in figure 1 (b), figure 1 (c), figure 1 (d), figure 1 (e) and figure 1 (f) In the face database corresponding to the five cases, 2DPCA, 2DLDA, 2DLPP, and 2DPLS respectively represent two-dimensional principal component analysis, two-dimensional discriminant analysis, two-dimensional local preservation mapping, two-dimensional partial least squares 2DNPLS, 2DNSPLS algorithm for testing. Experimental results such as Figure 3-7 shown.
[0097] Such as image 3 shown, at 9% occlusion of the figure 1 In (b), 2DLDA performs the worst when there are fewer training samples, followed by 2DLPP. 2DNSPLS algorithm shows better recognition rate and robustness under each training sample. The recognition rate of 2DNPLS algorithm decreases with the occurrence of occlusion, and other algorithms are greatly affected. Both 2DLDA and 2DLPP are greatly affected when the sample size is small.
[0098] Compare image 3 , 4 , 5, that is, in the fa...
Embodiment 3
[0100] Embodiment 3: Adopt the experiment on PIE face bank [face bank is the face bank that includes posture (pose), illumination (illumination) and expression (exprsssion) change that Carnegie Mellon University establishs] it includes from 68 people The 40,000 photos of each person have photos under 13 pose conditions, 43 lighting conditions and 4 expressions. In this embodiment, 45 photos of each person are selected as the total sample of the experiment. Figure 8 There are 45 pictures of 2 of them.
[0101] Select the first 5, 10, 15, 20, 25, 30, 35, and 40 pictures of each person for training, and the remaining pictures for testing. Experimental results such as Figure 9 shown.
[0102] Depend on Figure 9 It can be seen that the recognition rate of 2DNSPLS is significantly better than other algorithms when the number of training samples is small, and the advantage decreases with the increase of the number of training samples, but it is still better than other algorith...
PUM
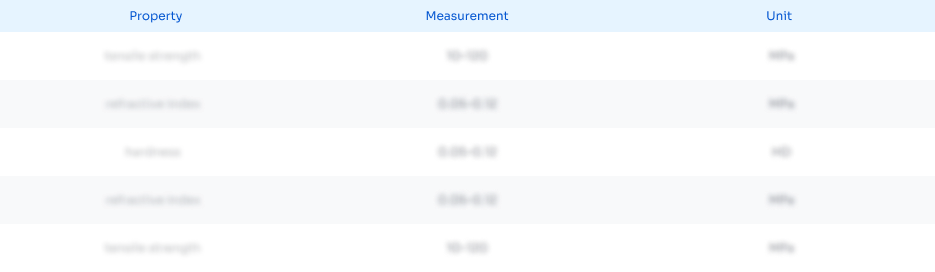
Abstract
Description
Claims
Application Information
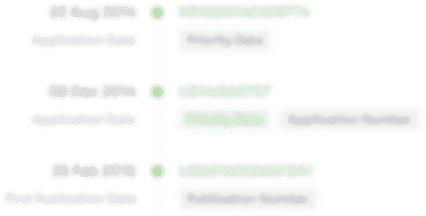
- R&D Engineer
- R&D Manager
- IP Professional
- Industry Leading Data Capabilities
- Powerful AI technology
- Patent DNA Extraction
Browse by: Latest US Patents, China's latest patents, Technical Efficacy Thesaurus, Application Domain, Technology Topic, Popular Technical Reports.
© 2024 PatSnap. All rights reserved.Legal|Privacy policy|Modern Slavery Act Transparency Statement|Sitemap|About US| Contact US: help@patsnap.com