MRI glioma segmentation method and system based on sparse Bayesian model and multi-map fusion
A sparse Bayesian and glioma technology, applied in the field of medical image processing, can solve problems such as low contrast, long processing time, and high computational consumption, and achieve precise segmentation and clear boundaries
- Summary
- Abstract
- Description
- Claims
- Application Information
AI Technical Summary
Problems solved by technology
Method used
Image
Examples
Embodiment 1
[0045] Such as figure 1 As shown, an MRI glioma segmentation method based on sparse Bayesian model and multi-atlas fusion, the method includes:
[0046] An MRI glioma segmentation method based on sparse Bayesian model and multi-atlas fusion, the method includes:
[0047] Step S1: Receive the MRI, perform preprocessing, and obtain an image in which all modalities of the MRI are co-registered to a unified anatomical template and have segmentation labels for auxiliary diagnosis;
[0048] The sparse Bayesian model is used to segment the different modalities of MRI, and the segmentation result labels of different modalities are obtained;
[0049] The multi-atlas fusion method was used to fuse the segmentation results of different modalities in MRI to obtain the final glioma segmentation results with clear boundaries.
[0050] In step S1 of one or more embodiments of the present disclosure, the specific steps of the preprocessing include:
[0051] The GLISTRboost algorithm is use...
Embodiment 2
[0083] According to an aspect of one or more embodiments of the present disclosure, there is provided a computer-readable storage medium. A computer-readable storage medium, wherein a plurality of instructions are stored, and the instructions are suitable for being loaded by a processor of a terminal device and executing the MRI glioma based on a sparse Bayesian model and multi-atlas fusion split method.
Embodiment 3
[0085] According to an aspect of one or more embodiments of the present disclosure, a terminal device is provided.
[0086] A terminal device, which includes a processor and a computer-readable storage medium, the processor is used to implement instructions; the computer-readable storage medium is used to store multiple instructions, and the instructions are suitable for being loaded by the processor and executing the described one An MRI Glioma Segmentation Method Based on Sparse Bayesian Model and Multi-Atlas Fusion.
[0087] These computer-executable instructions, when executed in a device, cause the device to perform the methods or processes described in accordance with various embodiments in the present disclosure.
[0088]In this embodiment, a computer program product may include a computer-readable storage medium carrying computer-readable program instructions for performing various aspects of the present disclosure. A computer readable storage medium may be a tangible...
PUM
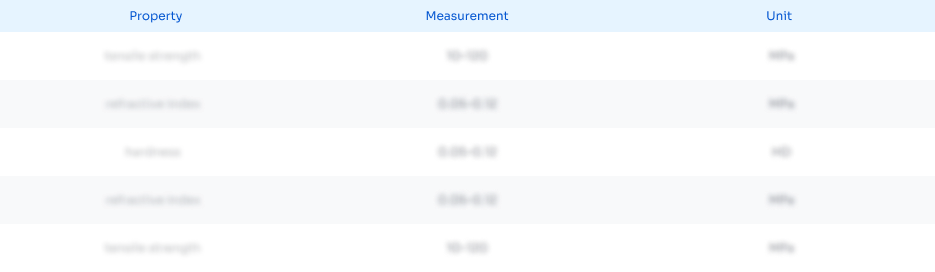
Abstract
Description
Claims
Application Information
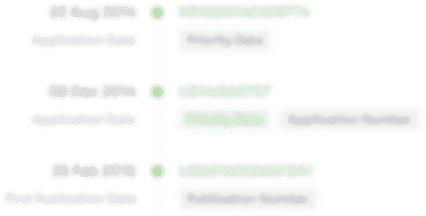
- R&D Engineer
- R&D Manager
- IP Professional
- Industry Leading Data Capabilities
- Powerful AI technology
- Patent DNA Extraction
Browse by: Latest US Patents, China's latest patents, Technical Efficacy Thesaurus, Application Domain, Technology Topic, Popular Technical Reports.
© 2024 PatSnap. All rights reserved.Legal|Privacy policy|Modern Slavery Act Transparency Statement|Sitemap|About US| Contact US: help@patsnap.com