Block-wise Compressive Sensing Reconstruction Method Based on Image Patch Clustering and Sparse Dictionary Learning
A block compressive sensing and sparse dictionary technology, applied in the field of image processing, can solve the problems of not being able to flexibly describe different features and not using the similarity of sub-image blocks.
- Summary
- Abstract
- Description
- Claims
- Application Information
AI Technical Summary
Problems solved by technology
Method used
Image
Examples
Embodiment Construction
[0044] The present invention will be described in further detail below in conjunction with the accompanying drawings. The specific implementation process of the present invention is as follows.
[0045] (1), divide an image into sub-image blocks, the size of the sub-image in this example is .
[0046] (2), for each sub-image block with The measurement rate is compressed and sampled to obtain the measurement ;
[0047] ,in is the first The pixel values of sub-image blocks, yes Randomly undersampled matrix, , yes The number of non-zero elements in , .
[0048] (3) Generate a value between 0 and 180 degrees black and white edge images in one direction, for all edge images The sub-image blocks are decomposed by PCA to generate direction PCA basis , and then select a DCT dictionary ,constitute The initial set-associated direction dictionary of direction bases, where yes matrix, in this example Set to 19.
[0049] (4), calculation an...
PUM
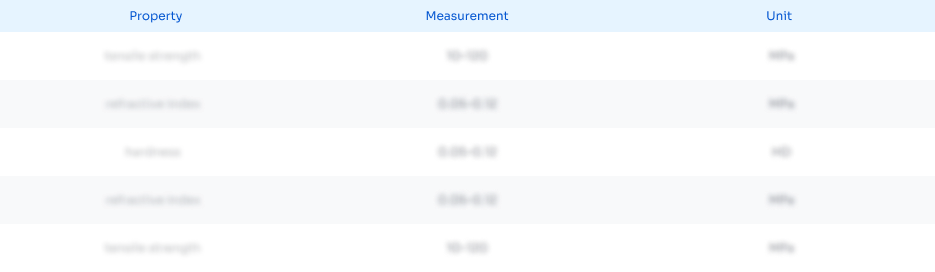
Abstract
Description
Claims
Application Information
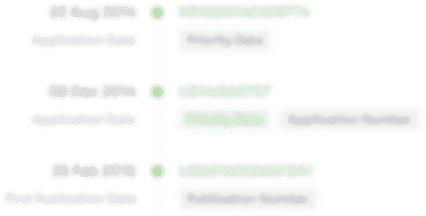
- R&D
- Intellectual Property
- Life Sciences
- Materials
- Tech Scout
- Unparalleled Data Quality
- Higher Quality Content
- 60% Fewer Hallucinations
Browse by: Latest US Patents, China's latest patents, Technical Efficacy Thesaurus, Application Domain, Technology Topic, Popular Technical Reports.
© 2025 PatSnap. All rights reserved.Legal|Privacy policy|Modern Slavery Act Transparency Statement|Sitemap|About US| Contact US: help@patsnap.com