Polarized SAR (synthetic aperture radar) image classification method based on depth PCA (principal component analysis) network and SVM (support vector machine)
A technology of PCA network and classification method, applied in the field of polarization SAR image classification based on deep principal component analysis network and SVM, can solve the problems that cannot meet the requirements, the characteristics are not enough to characterize, and the scattering mechanism of ground objects cannot be fully described. Achieve the effect of improving classification results and classification accuracy, and improving classification results
- Summary
- Abstract
- Description
- Claims
- Application Information
AI Technical Summary
Problems solved by technology
Method used
Image
Examples
Embodiment Construction
[0037] refer to figure 1 , the specific implementation steps of the present invention are as follows:
[0038] Step 1, read in a polarimetric SAR image to be classified;
[0039] Step 2, using the refined polarization LEE filtering method for the polarimetric SAR image to be classified to obtain the filtered polarimetric SAR image;
[0040] Filter the read-in polarimetric SAR images to be classified to achieve speckle suppression.
[0041] Preferably, the filtering method adopted is the refined polarization LEE filtering method, and the size of the filtering window is set to 7×7.
[0042] In addition, filtering methods that can also be used include polarization whitening filtering, box car filtering, and filtering methods based on unsupervised classification.
[0043] Step 3, for the covariance matrix C representing each pixel in the filtered polarimetric SAR image, extract the data distribution characteristic parameter α; for the covariance matrix C representing each pixel...
PUM
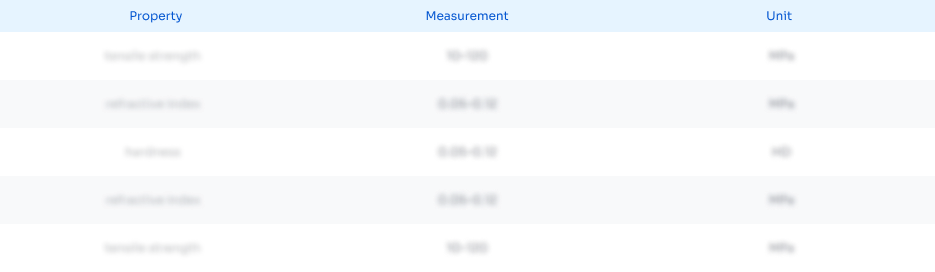
Abstract
Description
Claims
Application Information
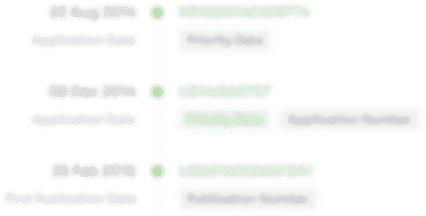
- R&D Engineer
- R&D Manager
- IP Professional
- Industry Leading Data Capabilities
- Powerful AI technology
- Patent DNA Extraction
Browse by: Latest US Patents, China's latest patents, Technical Efficacy Thesaurus, Application Domain, Technology Topic, Popular Technical Reports.
© 2024 PatSnap. All rights reserved.Legal|Privacy policy|Modern Slavery Act Transparency Statement|Sitemap|About US| Contact US: help@patsnap.com