Adaptive threshold value iterative reconstruction method for distributed compressed sensing
An adaptive threshold and compressed sensing technology, applied in the field of signal processing, can solve the problems of long reconstruction time, large reconstruction error, and constant step size of the simultaneous orthogonal matching pursuit method, and achieve step size adaptive iteration threshold, The effect of less reconstruction time and reduction of reconstruction errors
- Summary
- Abstract
- Description
- Claims
- Application Information
AI Technical Summary
Problems solved by technology
Method used
Image
Examples
specific Embodiment approach 1
[0026] combine figure 1 The specific implementation mode 1 is described as follows:
[0027] Step 1. Input: perception matrix A, observation value Y, maximum iteration number inter_max, iteration termination threshold error, joint sparsity s, iteration initial value x k ;
[0028] Initialization: number of iterations inter=1, parameters τ and μ, support set S={||(A T Y) i || 2 the largest s indices, i∈[1,N]};
[0029] The perception matrix A and the observed value Y are specifically as follows: Observing J signals to obtain the observed value Y: Y=[y 1 the y 2 … y J ], the y j is the observed value of signal j, M is the observed value y j The number of data, j∈[1, J], N is the length of signal acquisition data.
[0030] Step 2. Calculate the adaptive step size τ and the adaptive threshold h, the specific calculation process:
[0031] 1) Calculation parameter rk, rk=sum((Ax k -Y).^2);
[0032] 2) Calculate the adaptive step size τ,
[0033] 3) Calculate ...
PUM
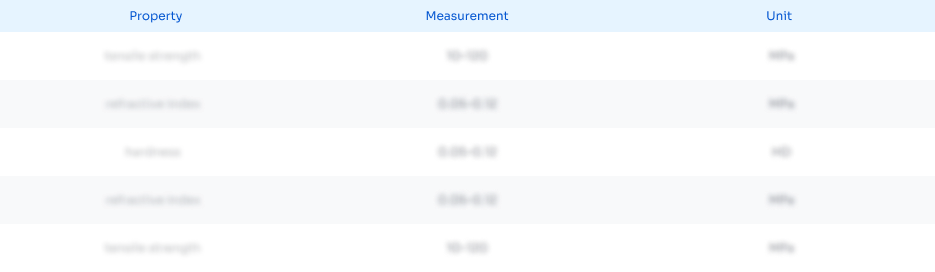
Abstract
Description
Claims
Application Information
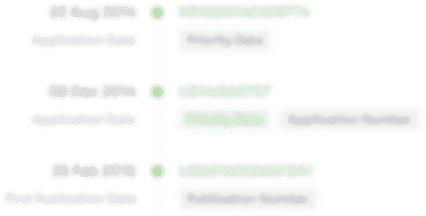
- R&D Engineer
- R&D Manager
- IP Professional
- Industry Leading Data Capabilities
- Powerful AI technology
- Patent DNA Extraction
Browse by: Latest US Patents, China's latest patents, Technical Efficacy Thesaurus, Application Domain, Technology Topic, Popular Technical Reports.
© 2024 PatSnap. All rights reserved.Legal|Privacy policy|Modern Slavery Act Transparency Statement|Sitemap|About US| Contact US: help@patsnap.com