Improved neural network model based on particle swarm optimization algorithm for data prediction method
A neural network model and particle swarm optimization technology, applied in the field of neural network models, can solve problems such as the difficulty of determining the number of neurons in the hidden layer and the impact of RBF neural network performance
- Summary
- Abstract
- Description
- Claims
- Application Information
AI Technical Summary
Problems solved by technology
Method used
Image
Examples
Embodiment 1
[0050] Embodiment 1: as attached figure 1 As shown, the improved neural network model based on the particle swarm optimization algorithm is used in the data prediction method, and the following steps are carried out:
[0051] Step 1: Representation of the data sample, using variables Denotes the i-th sample, using Indicates the t-th component of the i-th sample;
[0052] Step 2: Data preprocessing, constructing a training sample set X based on data samples train ={x 1 ,x 2 ,...,x N} and test sample set X test ={x 1 ,x 2 ,...,x K};
[0053] Step 3: Initialize the parameters of the RBF neural network, and determine the number N of neurons in the input layer according to the dimension M of the training sample set input =M, determine the number of neurons in the output layer N according to the dimensionality of the data to be predicted output =P;
[0054] Step 4: Use the binary particle swarm optimization algorithm to determine the number of hidden layer neurons and...
Embodiment 2
[0064] Step 14: Use the RBF neural network model for data prediction, use the test sample set as the input sample of the RBF neural network model, and use the established RBF neural network model to predict unknown data. The hidden layer of the RBF network model uses Radial basis kernel function, the formula of radial basis kernel function is x i Indicates the input sample, c i Indicates the center of the kernel function of the i-th neuron in the hidden layer, σ indicates the width of the kernel function, using the formula Calculate the predicted output value of the RBF neural network model, that is, the predicted value for unknown data. Embodiment 2: The difference from the above embodiment is that step 4 includes the following sub-steps:
[0065] Sub-step 401: Binary particle swarm optimization algorithm parameter initialization, the number of initialized particle swarms is N swarm Represents; randomly initialize the position of the particle swarm with said, among the...
Embodiment 3
[0077] Embodiment 3: The difference from the above embodiment is that step 8 includes the following sub-steps:
[0078] Sub-step 801: construct a kd tree (k-dimensional tree) according to the k-nearest neighbor algorithm (k-nearest neighbor, k-NN) and the values of each dimension of the particles in the particle swarm;
[0079] Sub-step 802: According to the kd tree search principle, find the k nearest neighbor particles of each particle, i.e. top(k) particles;
[0080] Sub-step 803: According to the fitness function values of the k nearest neighbor particles obtained from the search, determine the optimal particle p in the neighborhood of each particle lbest ;
[0081] Sub-step 804: k=k+1, that is, the neighborhood of each particle expands the range of one particle during the next iteration, until the value of k is equal to the size of the particle swarm, k=N l-swarm .
PUM
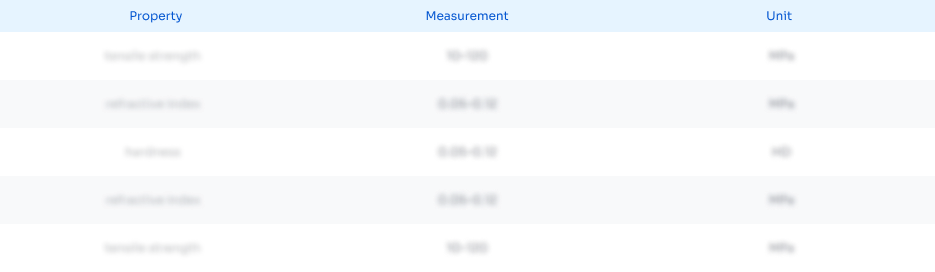
Abstract
Description
Claims
Application Information
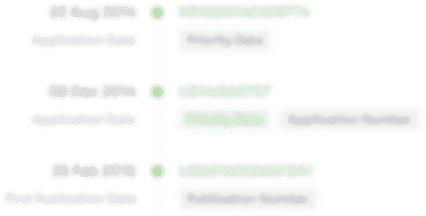
- R&D
- Intellectual Property
- Life Sciences
- Materials
- Tech Scout
- Unparalleled Data Quality
- Higher Quality Content
- 60% Fewer Hallucinations
Browse by: Latest US Patents, China's latest patents, Technical Efficacy Thesaurus, Application Domain, Technology Topic, Popular Technical Reports.
© 2025 PatSnap. All rights reserved.Legal|Privacy policy|Modern Slavery Act Transparency Statement|Sitemap|About US| Contact US: help@patsnap.com