Non-reference image quality evaluation method based on discrete cosine transform and sparse representation
A discrete cosine transform and sparse representation technology, applied in the field of image processing, can solve the problems of difficult algorithm design and implementation, long time consumption, and inability to accurately simulate the mapping relationship.
- Summary
- Abstract
- Description
- Claims
- Application Information
AI Technical Summary
Problems solved by technology
Method used
Image
Examples
Embodiment Construction
[0032] Below in conjunction with accompanying drawing, specific implementation steps and effects of the present invention are described in further detail:
[0033] refer to figure 1 , the implementation steps of the present invention are as follows:
[0034] Step 1, extract the natural scene statistical features f of the grayscale image I on the first scale s ;
[0035] (1a) Read in a grayscale image I, decompose the grayscale image I into 5*5 overlapping image blocks, perform discrete cosine transform on each image block, and remove the direct current of discrete cosine transform coefficients weight;
[0036] (1b) Use the generalized Gaussian distribution model to fit the discrete cosine transform coefficients of each image block after removing the DC component to obtain the shape factor γ of each image block, and take the mean value of the shape factor γ of all image blocks as the first Statistical features of natural scenes at scales f s,1 The first element f of s,1,1...
PUM
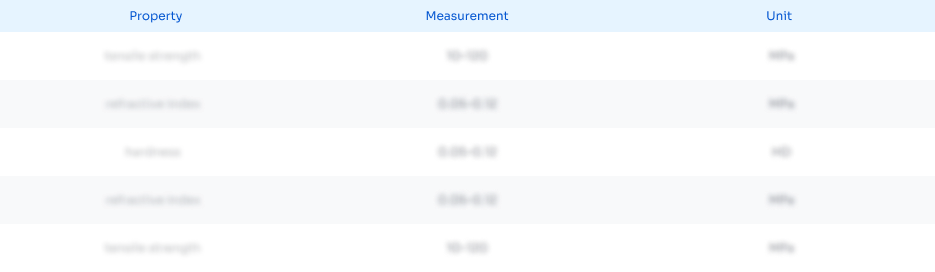
Abstract
Description
Claims
Application Information
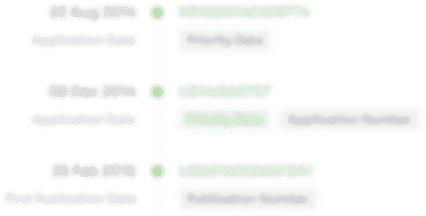
- R&D Engineer
- R&D Manager
- IP Professional
- Industry Leading Data Capabilities
- Powerful AI technology
- Patent DNA Extraction
Browse by: Latest US Patents, China's latest patents, Technical Efficacy Thesaurus, Application Domain, Technology Topic, Popular Technical Reports.
© 2024 PatSnap. All rights reserved.Legal|Privacy policy|Modern Slavery Act Transparency Statement|Sitemap|About US| Contact US: help@patsnap.com