Image classification method based on kernel principal component analysis network
A technology of kernel principal component analysis and classification methods, applied in the field of digital images, can solve the problems of very sensitive training data noise, loss of nonlinear energy in high dimensions, lack of normalization of functions, etc.
- Summary
- Abstract
- Description
- Claims
- Application Information
AI Technical Summary
Problems solved by technology
Method used
Image
Examples
Embodiment Construction
[0170] The technical solution of the present invention will be described in detail below with reference to the drawings and embodiments.
[0171] Such as Figure 1-4 As shown, in the present invention, the image classification method based on the kernel principal component analysis network constructs a new image feature extraction structure by cascading two layers of kernel principal component analysis filters, which is called the kernel principal component analysis network, and the constructed The kernel PCA network was tested, including the following steps.
[0172] Step 1: Establish the first layer of the kernel PCA network
[0173] Step 1.1: Randomly select N from the image database of N size m×n 1 as a training image database; use a size k 1 ×k 2 The slider for iterates over each training image in the training image database for each pixel of is the set of real numbers, k 1 and k 2 are odd, and 01 ≤m,02 ≤n, each image has a total of mn pixels; for each pixel, k ...
PUM
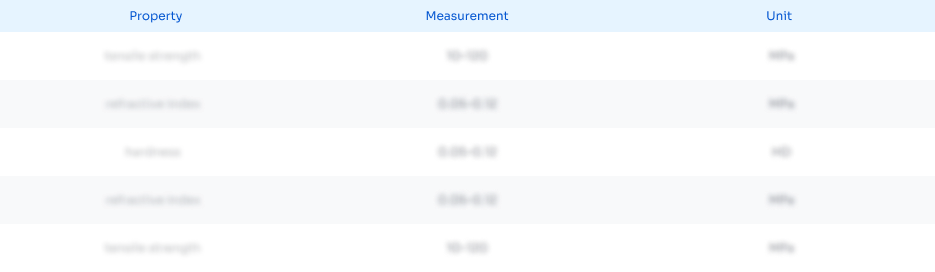
Abstract
Description
Claims
Application Information
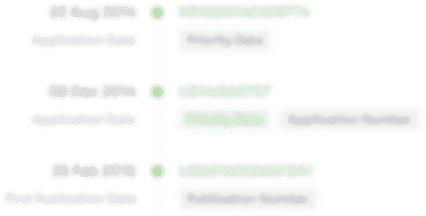
- R&D Engineer
- R&D Manager
- IP Professional
- Industry Leading Data Capabilities
- Powerful AI technology
- Patent DNA Extraction
Browse by: Latest US Patents, China's latest patents, Technical Efficacy Thesaurus, Application Domain, Technology Topic, Popular Technical Reports.
© 2024 PatSnap. All rights reserved.Legal|Privacy policy|Modern Slavery Act Transparency Statement|Sitemap|About US| Contact US: help@patsnap.com