A method for image super-resolution based on SAE and sparse representation
A super-resolution and sparse representation technology, applied in the field of image processing, can solve problems such as data overfitting, difficulty in dictionary training, and increased dimension of feature space, so as to avoid time-consuming, labor-intensive, adverse effects, and feature extraction single effect
- Summary
- Abstract
- Description
- Claims
- Application Information
AI Technical Summary
Problems solved by technology
Method used
Image
Examples
Embodiment Construction
[0022] The preferred embodiments of the present invention will be described in detail below with reference to the accompanying drawings.
[0023] figure 1 It is an overall flowchart of the method of the present invention. As shown in the figure, the implementation process of image super-resolution technology based on sparse representation is divided into two processes, namely, the offline training phase and the reconstruction testing phase. The purpose of the offline training phase is to build high- and low-resolution dictionary pairs that describe the relationship between high- and low-resolution image features; the reconstruction test phase is to use the relationship between dictionary pairs to estimate high-resolution images from low-resolution test images input by users , to make up for the high-frequency detail information missing in the low-resolution image during the sampling process. During off-line dictionary training, the present invention has used the image feature...
PUM
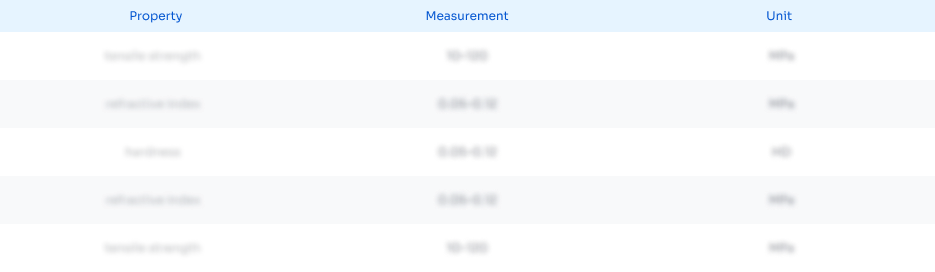
Abstract
Description
Claims
Application Information
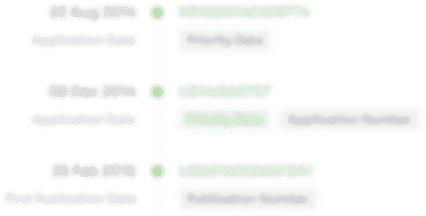
- R&D
- Intellectual Property
- Life Sciences
- Materials
- Tech Scout
- Unparalleled Data Quality
- Higher Quality Content
- 60% Fewer Hallucinations
Browse by: Latest US Patents, China's latest patents, Technical Efficacy Thesaurus, Application Domain, Technology Topic, Popular Technical Reports.
© 2025 PatSnap. All rights reserved.Legal|Privacy policy|Modern Slavery Act Transparency Statement|Sitemap|About US| Contact US: help@patsnap.com