Cellular network base station state time-varying model establishing method based on Bayesian network
A Bayesian network and model building technology, which is applied to the establishment of time-varying statistical models of cellular network base station states. The field of cellular network base station state time-varying model establishment based on Bayesian structure learning can solve adaptive adjustment, Bayesian The computational complexity of the Si network structure is high, but the computational complexity is low, and the effect of low complexity is achieved.
- Summary
- Abstract
- Description
- Claims
- Application Information
AI Technical Summary
Problems solved by technology
Method used
Image
Examples
Embodiment 1
[0053] In the field of modern mobile communications, the data transmission of mobile primary users in the actual cellular network is affected by the dynamic change of the base station switch state. The prediction of the time-varying law of the base station state in the cellular network can reduce the collision of mobile user services and increase the reliability of data transmission. To study the prediction of the time-varying law of the base station state needs to reduce the complexity and increase the reliability. In this regard, the typical Bayesian network structure can be used to reduce the complexity of learning the Bayesian structure to reduce the state of the cellular network base station. The complexity of the time-varying model.
[0054] In order to achieve effective low-complexity prediction of cellular network base station status, refer to figure 1 , the present invention proposes a method for establishing a time-varying model of a cellular network base station sta...
Embodiment 2
[0060] The cellular network base station state time-varying model establishment method based on Bayesian network is the same as embodiment 1, wherein the establishment of Bayesian network model in step (2) includes the following steps:
[0061] 2.1. Define the nodes of the Bayesian network:
[0062] The base station state f perceived by the secondary sensing device i,t Defined as a Bayesian network node, the nodes are ordered in the time domain, and the state of the i-th base station observed by the secondary sensing device at time t, f i,t ∈O={0,1}, O represents the state set, 0 and 1 represent the base station off (off) and on (on) state, respectively, i∈M={1,2,...M}, M represents the observed base station Sequence, t∈T={1,2,...T}, T represents the observation time sequence. In this example, the secondary sensing device perceives the state of the base station every 1 second, and the state of the base station observed by the secondary sensing device is randomly generated by...
Embodiment 3
[0069] The cellular network base station state time-varying model establishment method based on Bayesian network is the same as embodiment 1 and embodiment 2, and its step 2.3 described obtains the conditional probability table by the learning of Bayesian network structure and comprises the following steps:
[0070] 2.3a Calculate the parameter matrix C from the first-order fully connected Bayesian network established in 2.1 and 2.2 above:
[0071]
[0072] In the formula, C[x,M-i+1] represents the element of row x and column M-i+1 of parameter matrix C, x=0,1,...,2 M -1, i={1,2,...M}; M represents the maximum value of the number of observed base stations; Indicates rounding down operation; \Indicates touch operation;
[0073]2.3b Calculate the operator parameter matrix F according to the parameter matrix C obtained above:
[0074] F = nom ( F ) / ( nom...
PUM
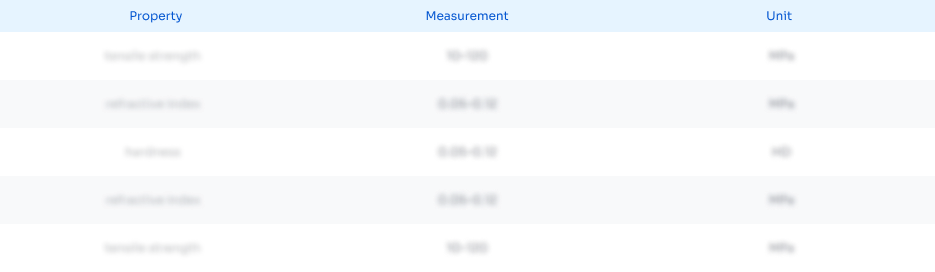
Abstract
Description
Claims
Application Information
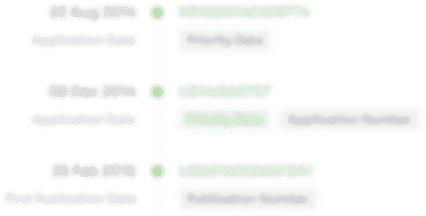
- R&D Engineer
- R&D Manager
- IP Professional
- Industry Leading Data Capabilities
- Powerful AI technology
- Patent DNA Extraction
Browse by: Latest US Patents, China's latest patents, Technical Efficacy Thesaurus, Application Domain, Technology Topic, Popular Technical Reports.
© 2024 PatSnap. All rights reserved.Legal|Privacy policy|Modern Slavery Act Transparency Statement|Sitemap|About US| Contact US: help@patsnap.com