Multiclass image classification method based on semi-supervised extreme learning machine
An extreme learning machine and semi-supervised learning technology, applied in computer parts, instruments, character and pattern recognition, etc., can solve the problems of low learning speed and low accuracy of image classification, and achieve high accuracy and robustness. Effect
- Summary
- Abstract
- Description
- Claims
- Application Information
AI Technical Summary
Problems solved by technology
Method used
Image
Examples
Embodiment Construction
[0035]The present invention will be further described below in conjunction with the accompanying drawings.
[0036] Such as figure 1 , figure 2 Shown is a multi-class image classification method based on semi-supervised extreme learning machine, which specifically includes the following:
[0037] (1) The number of initialization experiments is K, i=1;
[0038] (2) Shuffle and rearrange the order of samples in the training sample set, take a certain proportion of samples (usually with a lower proportion) at the top as the labeled sample set L, and take a certain proportion of samples after that (usually with a higher proportion ) is used as the unlabeled sample set U, and the remaining samples are used as the test sample set T, and the number of samples in the labeled sample set L is smaller than the number of samples in the unlabeled sample set U;
[0039] (3) The number of initialization iterations is M, j=1;
[0040] (4) Construct N different training subsets based on t...
PUM
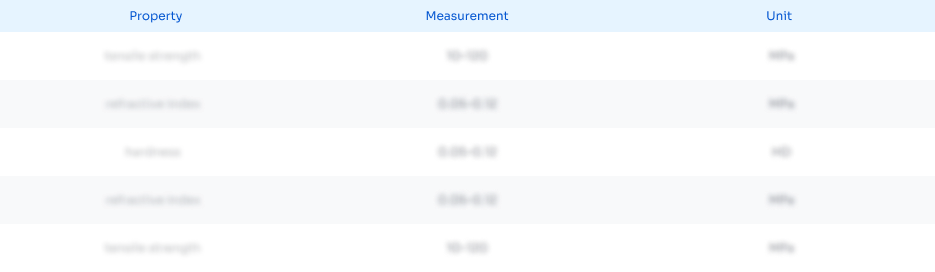
Abstract
Description
Claims
Application Information
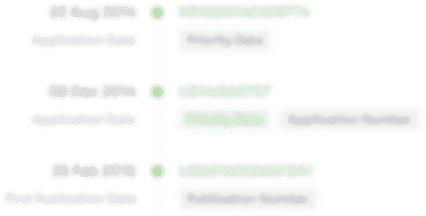
- R&D
- Intellectual Property
- Life Sciences
- Materials
- Tech Scout
- Unparalleled Data Quality
- Higher Quality Content
- 60% Fewer Hallucinations
Browse by: Latest US Patents, China's latest patents, Technical Efficacy Thesaurus, Application Domain, Technology Topic, Popular Technical Reports.
© 2025 PatSnap. All rights reserved.Legal|Privacy policy|Modern Slavery Act Transparency Statement|Sitemap|About US| Contact US: help@patsnap.com