Image super-resolution reconstruction method based on representational learning and neighbor constraint embedding
A super-resolution and neighborhood technology, applied in the field of image processing, can solve the problems of general reconstructed image quality, missing neighbors, and inaccurate extraction, etc., to overcome poor compatibility, improve restoration effect, detail and texture information Good results
- Summary
- Abstract
- Description
- Claims
- Application Information
AI Technical Summary
Problems solved by technology
Method used
Image
Examples
Embodiment 1
[0030] The present invention is an image super-resolution reconstruction method based on representation learning and neighborhood constraint embedding, see figure 1 , the present invention includes the following steps to image super-resolution reconstruction:
[0031] Step 1: Input the training sample image pair, and use the low-resolution training sample image to learn to construct a low-resolution image block dictionary with a scale of 10W And use the high-resolution training sample images to learn to construct a dictionary with a size of N and low-resolution image blocks The corresponding high-resolution image patch dictionary The training image pairs used are standard natural images commonly used in the field of image processing, see figure 2 , image 3 , Figure 4 .
[0032] Step 2: Input the low-resolution test image Y to be super-resolution reconstructed, and divide it into blocks in an overlapping manner to obtain a low-resolution image block set For each bl...
Embodiment 2
[0040] The image super-resolution reconstruction method based on representation learning and neighborhood constraint embedding is the same as in Embodiment 1. Step 1 and step 2 in the present invention both involve the process of feature extraction, and both use a deep sparse autoencoder network to learn the characteristics of image blocks. Therefore, it is summarized as follows:
[0041] Wherein step 1 trains the training sample image pairs, including the following steps:
[0042] 1a) Input the training image pair, block the high-resolution and low-resolution images respectively, and obtain the low-resolution image block set and the corresponding set of high-resolution image patches The training image pairs used are standard natural images commonly used in the field of image processing, see figure 2 , image 3 , Figure 4 ;
[0043] 1b) For low-resolution training image patch set X sp , through the training of deep sparse autoencoder network to obtain cascaded featur...
Embodiment 3
[0050] The image super-resolution reconstruction method based on representation learning and neighborhood constraint embedding is the same as that in Embodiment 1-2.
[0051] Wherein the pre-selected feature block neighbor described in step 3 includes the following steps:
[0052] 3a) For an input low-resolution image feature block Compute feature blocks Dictionaries with low-resolution image patches the Euclidean distance;
[0053] 3b) Select the first K small blocks with the smallest distance as The preselected feature neighbors of yes In the low-resolution image patch dictionary X s The initial neighborhood in .
[0054] In the present invention, K=100 training low-resolution image blocks are selected as initial neighbors, and these 100 low-resolution image blocks are the most similar to the input feature block and have a fixed size. Too many neighbors will increase the complexity of the algorithm and slow down the operation speed, and too few neighbors will n...
PUM
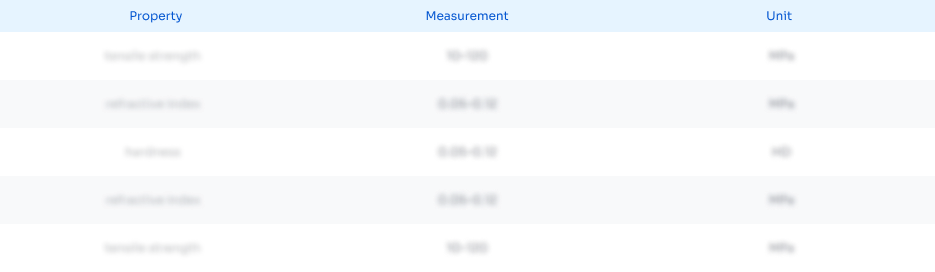
Abstract
Description
Claims
Application Information
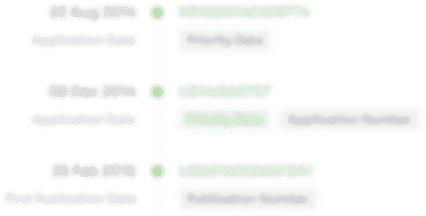
- R&D Engineer
- R&D Manager
- IP Professional
- Industry Leading Data Capabilities
- Powerful AI technology
- Patent DNA Extraction
Browse by: Latest US Patents, China's latest patents, Technical Efficacy Thesaurus, Application Domain, Technology Topic, Popular Technical Reports.
© 2024 PatSnap. All rights reserved.Legal|Privacy policy|Modern Slavery Act Transparency Statement|Sitemap|About US| Contact US: help@patsnap.com