Depth convolution neural network acceleration and compression method based on parameter quantification
A deep convolution and neural network technology, applied in the field of image processing, can solve the problem that the acceleration or compression effect of the network model needs to be studied, and the acceleration and compression of the convolutional neural network are not considered at the same time.
- Summary
- Abstract
- Description
- Claims
- Application Information
AI Technical Summary
Problems solved by technology
Method used
Image
Examples
Embodiment Construction
[0013] The method for accelerating and compressing a deep convolutional neural network based on parameter quantization provided by an embodiment of the present invention will be described in detail below with reference to the accompanying drawings.
[0014] figure 1 The flow chart of the method for accelerating and compressing the deep convolutional neural network based on parameter quantization provided by the embodiment of the present invention.
[0015] refer to figure 1 , in step S101, quantize the parameters of the deep convolutional neural network to obtain a plurality of sub-codebooks and index values corresponding to the plurality of sub-codebooks.
[0016] In step S102, the feature map of the output of the deep convolutional neural network is obtained according to the plurality of sub-codebooks and index values respectively corresponding to the plurality of sub-codebooks.
[0017] Here, the deep convolutional neural network includes multiple convolutional layers...
PUM
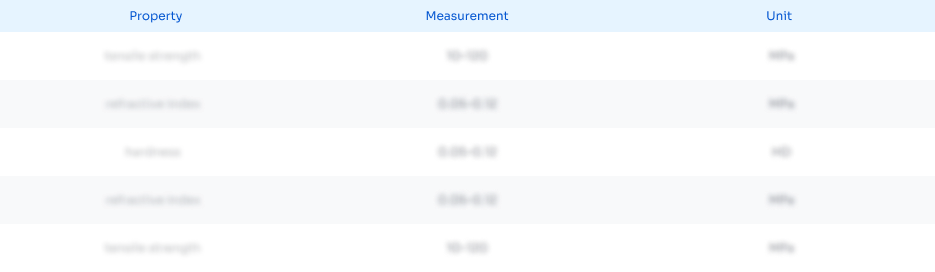
Abstract
Description
Claims
Application Information
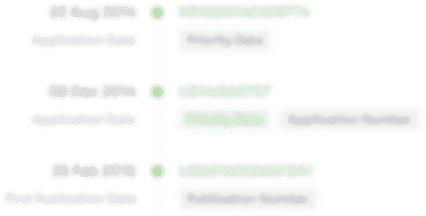
- R&D Engineer
- R&D Manager
- IP Professional
- Industry Leading Data Capabilities
- Powerful AI technology
- Patent DNA Extraction
Browse by: Latest US Patents, China's latest patents, Technical Efficacy Thesaurus, Application Domain, Technology Topic, Popular Technical Reports.
© 2024 PatSnap. All rights reserved.Legal|Privacy policy|Modern Slavery Act Transparency Statement|Sitemap|About US| Contact US: help@patsnap.com