Attack detection method and system
An attack detection and detection technology, applied in the Internet field, can solve the problems of lower detection efficiency and low detection rate of unknown attacks
- Summary
- Abstract
- Description
- Claims
- Application Information
AI Technical Summary
Problems solved by technology
Method used
Image
Examples
Embodiment Construction
[0047] The following will clearly and completely describe the technical solutions in the embodiments of the present invention with reference to the accompanying drawings in the embodiments of the present invention. Obviously, the described embodiments are only some, not all, embodiments of the present invention. Based on the embodiments of the present invention, all other embodiments obtained by persons of ordinary skill in the art without making creative efforts belong to the protection scope of the present invention.
[0048] In order to solve the problems in the prior art, an embodiment of the present invention provides an attack detection method and system. An attack detection method provided by an embodiment of the present invention is firstly introduced below.
[0049] Pre-establish a preset number of detection models related to HTTP requests.
[0050] figure 1 A schematic flowchart of an attack detection method provided by an embodiment of the present invention may in...
PUM
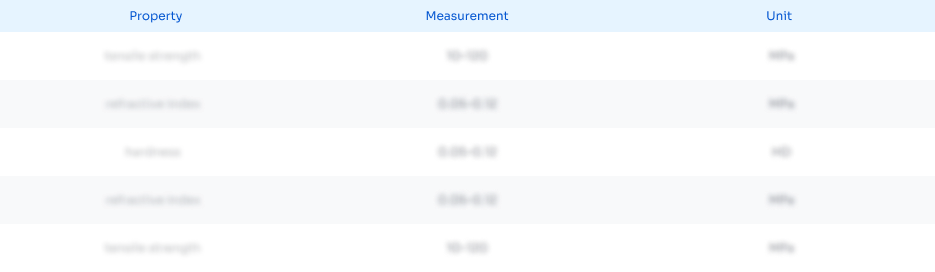
Abstract
Description
Claims
Application Information
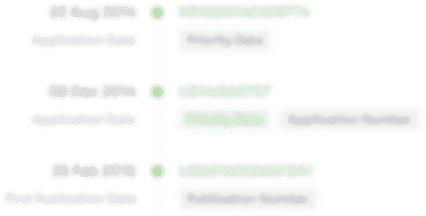
- R&D
- Intellectual Property
- Life Sciences
- Materials
- Tech Scout
- Unparalleled Data Quality
- Higher Quality Content
- 60% Fewer Hallucinations
Browse by: Latest US Patents, China's latest patents, Technical Efficacy Thesaurus, Application Domain, Technology Topic, Popular Technical Reports.
© 2025 PatSnap. All rights reserved.Legal|Privacy policy|Modern Slavery Act Transparency Statement|Sitemap|About US| Contact US: help@patsnap.com