Streetscape semantic annotation method based on convolutional neural network and semantic transfer conjunctive model
A technology of convolutional neural network and semantic transfer, which is applied in the field of street view labeling based on the joint model of convolutional neural network and semantic transfer, can solve the problems of unbalanced data sets, inability to extract richer and more differentiated target features, etc. , to achieve the effect of improving the labeling results and labeling accuracy
- Summary
- Abstract
- Description
- Claims
- Application Information
AI Technical Summary
Problems solved by technology
Method used
Image
Examples
Embodiment Construction
[0030] Now in conjunction with embodiment, accompanying drawing, the present invention will be further described:
[0031] The present invention proposes a street view labeling method based on a joint model of convolutional neural network and semantic transfer. Specifically, the algorithm improves the accuracy of street view labeling by extracting richer and more differentiated target features, combined with contextual information in the scene. In order to optimize the time performance, the invention transforms the pixel-by-pixel labeling problem into a superpixel labeling problem. Its technical solution includes two modules: deep feature extraction and soft-limited semantic transfer.
[0032] Feature extraction:
[0033] 1. Super pixel processing. First, the image is over-segmented into a certain number of superpixels, and the a priori information of the position of the superpixels in the original image is retained.
[0034] 2. Deep model training. Perform specific super...
PUM
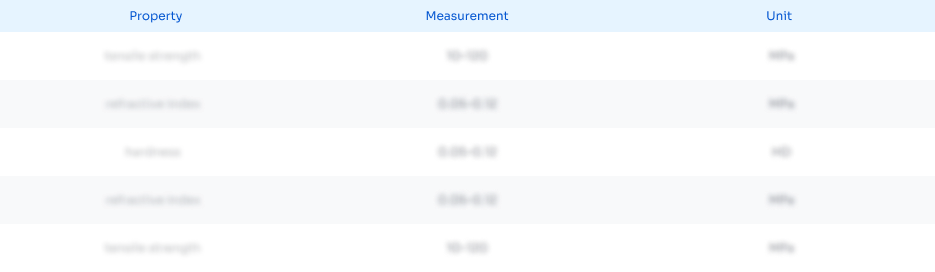
Abstract
Description
Claims
Application Information
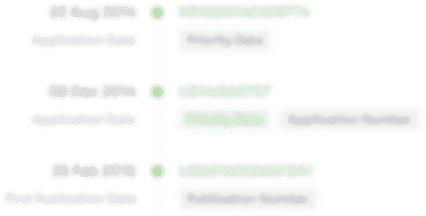
- R&D Engineer
- R&D Manager
- IP Professional
- Industry Leading Data Capabilities
- Powerful AI technology
- Patent DNA Extraction
Browse by: Latest US Patents, China's latest patents, Technical Efficacy Thesaurus, Application Domain, Technology Topic, Popular Technical Reports.
© 2024 PatSnap. All rights reserved.Legal|Privacy policy|Modern Slavery Act Transparency Statement|Sitemap|About US| Contact US: help@patsnap.com