Multi-feature extraction and fusion intelligent fault diagnosis method
A fault diagnosis and multi-feature technology, applied in the direction of instruments, character and pattern recognition, computer components, etc., can solve the problems of isolated feature extraction and fault recognition, few fault samples, and consume a lot of time, so as to reduce training time and improve the ability to reduce dependence on
- Summary
- Abstract
- Description
- Claims
- Application Information
AI Technical Summary
Problems solved by technology
Method used
Image
Examples
Embodiment Construction
[0037] In order to make the purpose, technical solutions and advantages of the present invention clearer, the present invention will be described in further detail below in conjunction with the accompanying drawings and embodiments; it should be understood that the specific embodiments described here are only used to explain the present invention and are not used limit the invention.
[0038] refer to figure 1 , an intelligent fault diagnosis method for multi-feature extraction and fusion, comprising the following steps:
[0039] (1) Use the data acquisition system and various sensors to collect data during the operation of mechanical equipment, such as vibration data and sound data;
[0040] (2) Intercept the original signal without any processing with a certain length, and divide it into training samples and test samples; make the frequency spectrum of each segment signal and normalize; the sample set (training, testing) is expressed as x n is the nth spectrum, d n is t...
PUM
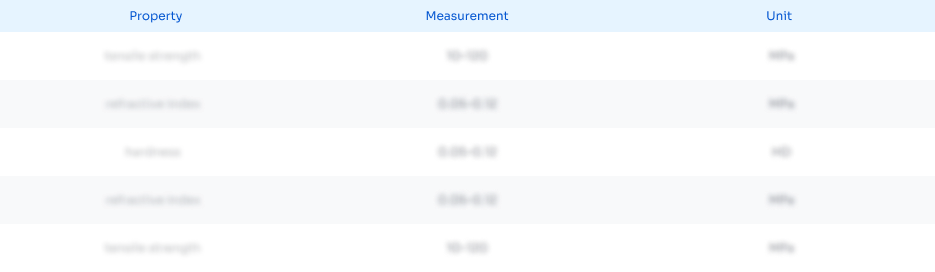
Abstract
Description
Claims
Application Information
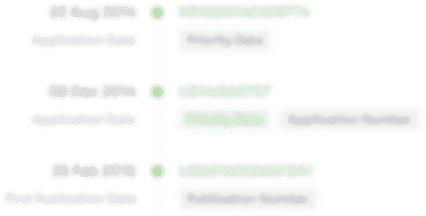
- R&D Engineer
- R&D Manager
- IP Professional
- Industry Leading Data Capabilities
- Powerful AI technology
- Patent DNA Extraction
Browse by: Latest US Patents, China's latest patents, Technical Efficacy Thesaurus, Application Domain, Technology Topic, Popular Technical Reports.
© 2024 PatSnap. All rights reserved.Legal|Privacy policy|Modern Slavery Act Transparency Statement|Sitemap|About US| Contact US: help@patsnap.com