A line fault identification method based on pole-line voltage machine learning discrimination mechanism
A technology of line fault and machine learning, applied in the direction of fault location, instrument, measuring electricity, etc., can solve problems such as difficult full-line quick movement, long transmission distance, etc.
- Summary
- Abstract
- Description
- Claims
- Application Information
AI Technical Summary
Problems solved by technology
Method used
Image
Examples
Embodiment 1
[0023] Example 1: The distance from the fault to the M terminal is 100km, and the transition resistance is 100Ω.
[0024] (1) Obtaining the output result of SVM according to steps (1)~(2) in the claims is 0;
[0025] (2) According to the step (3) in the claim, it is judged as a line fault.
Embodiment 2
[0026] Example 2: The fault distance is 400km from the M terminal, and the transition resistance is 100Ω.
[0027] (1) Obtaining the output result of SVM according to steps (1)~(2) in the claims is 0;
[0028] (2) According to the step (3) in the claim, it is judged as a line failure.
Embodiment 3
[0029] Embodiment 3: The distance from the fault to the M terminal is 1000km, and the transition resistance is 100Ω.
[0030] (1) According to the steps (1)~(2) in the claims, the output result of obtaining the SVM is 0;
[0031] (2) According to the step (3) in the claim, it is judged as a line failure.
PUM
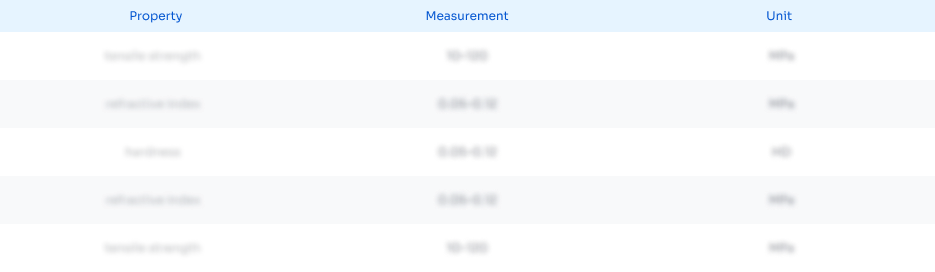
Abstract
Description
Claims
Application Information
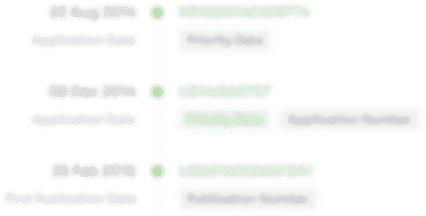
- R&D
- Intellectual Property
- Life Sciences
- Materials
- Tech Scout
- Unparalleled Data Quality
- Higher Quality Content
- 60% Fewer Hallucinations
Browse by: Latest US Patents, China's latest patents, Technical Efficacy Thesaurus, Application Domain, Technology Topic, Popular Technical Reports.
© 2025 PatSnap. All rights reserved.Legal|Privacy policy|Modern Slavery Act Transparency Statement|Sitemap|About US| Contact US: help@patsnap.com