Video semantic analysis method
A semantic analysis and video technology, applied in the direction of instruments, biological neural network models, character and pattern recognition, etc., can solve the problems of slow convergence of convolutional neural networks and failure to obtain labels
- Summary
- Abstract
- Description
- Claims
- Application Information
AI Technical Summary
Problems solved by technology
Method used
Image
Examples
Embodiment Construction
[0060] The technical solutions of the present invention will be described in further detail below in conjunction with the accompanying drawings and specific embodiments.
[0061] refer to figure 1 and figure 2 As shown, according to a preferred embodiment of the present invention, the video semantic analysis method based on the topology model pre-training convolutional neural network includes the following steps S1: preprocessing the video training set, and constructing a sparse linear decoder; S2: adding topology Establish a topological linear decoder with characteristic constraints, and divide the video training set into image blocks to train the topological linear decoder; S3: Use the parameters of the trained topological linear decoder as the initial parameters of the convolutional layer in the convolutional neural network; S4: Use the multi-fold cross-validation method and establish a key frame set based on the video training set to fine-tune the convolutional neural ne...
PUM
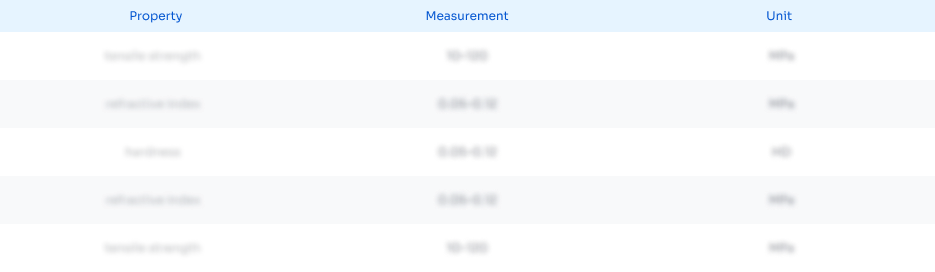
Abstract
Description
Claims
Application Information
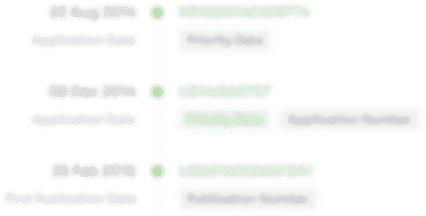
- R&D Engineer
- R&D Manager
- IP Professional
- Industry Leading Data Capabilities
- Powerful AI technology
- Patent DNA Extraction
Browse by: Latest US Patents, China's latest patents, Technical Efficacy Thesaurus, Application Domain, Technology Topic.
© 2024 PatSnap. All rights reserved.Legal|Privacy policy|Modern Slavery Act Transparency Statement|Sitemap