Multimodal Manifold Embedding Method for Zero-Shot Learning
A sample learning, multi-modal technology, applied in computer parts, character and pattern recognition, instruments, etc., can solve the problem that zero-sample learning cannot be applied, and achieve the effect of simple and feasible practicability, improved classification effect, and fast speed.
- Summary
- Abstract
- Description
- Claims
- Application Information
AI Technical Summary
Problems solved by technology
Method used
Image
Examples
Embodiment Construction
[0024] The multimodal manifold embedding method for zero-shot learning of the present invention will be described in detail below with reference to the embodiments and the accompanying drawings.
[0025] The multi-modal manifold embedding method for zero-sample learning of the present invention is mainly based on the traditional least squares regression method, adding local manifold constraints, and integrating the manifold information between samples of the same mode in the Maintain before and after mapping, and add intra-class compactness and inter-class separation to the objective function, so that the mapped samples are close to the same kind of samples in the corresponding mode, and separated from the different class samples in the corresponding mode. The method proposed in the present invention will be described below using the image mode and the text mode as two specific modes.
[0026] The image feature matrix of the training sample uses X=[X 1 ,...,X n ] means, wher...
PUM
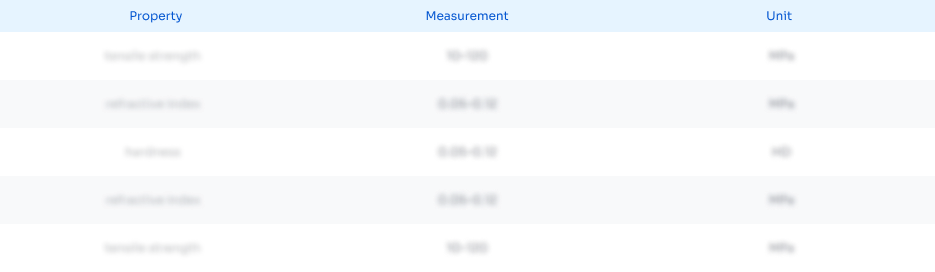
Abstract
Description
Claims
Application Information
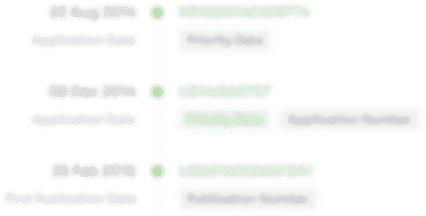
- R&D Engineer
- R&D Manager
- IP Professional
- Industry Leading Data Capabilities
- Powerful AI technology
- Patent DNA Extraction
Browse by: Latest US Patents, China's latest patents, Technical Efficacy Thesaurus, Application Domain, Technology Topic, Popular Technical Reports.
© 2024 PatSnap. All rights reserved.Legal|Privacy policy|Modern Slavery Act Transparency Statement|Sitemap|About US| Contact US: help@patsnap.com