Global-local optimization model based on multistage convolution neural network and significant detection algorithm
A convolutional neural network and local optimization technology, applied in computing, computer components, character and pattern recognition, etc., can solve problems such as dependence on computing speed, limited running speed, and speed of segmentation algorithms
- Summary
- Abstract
- Description
- Claims
- Application Information
AI Technical Summary
Problems solved by technology
Method used
Image
Examples
Embodiment Construction
[0034] The present invention provides a saliency detection algorithm based on a multi-level convolutional neural network. The global-local optimization model (GE-RM) based on the convolutional neural network is composed of a global estimation model (GEM) and a local optimization model ( RfM) composition;
[0035] The global estimation model has two output paths, the initialization branch path and the main path.
[0036] The initialization branch path of the global estimation model consists of a cascade of parts A and B, and part A consists of seven convolutional layers and three pooling layers. The preferred connection order is:
[0037] conv1-pool1-conv2-conv3-conv4-pool2-conv5-conv6-pool3-conv7,
[0038] Among them, conv1 is the input;
[0039] Part B consists of two cascaded fully connected layers (FC), of which the fully connected layer at the end is used as the output layer; preferably, the output layer has 4096 output units, which can form a 64x64 saliency map.
[004...
PUM
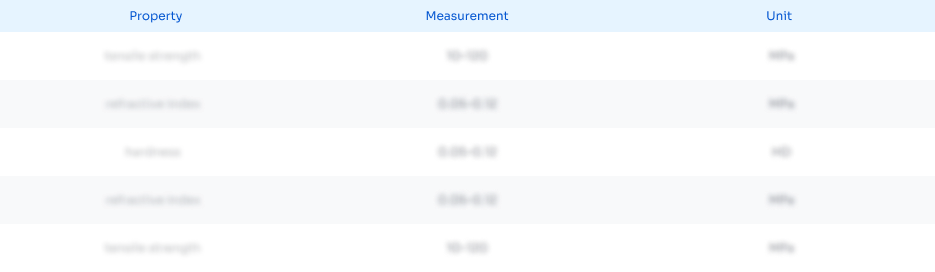
Abstract
Description
Claims
Application Information
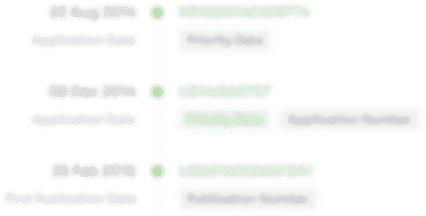
- R&D
- Intellectual Property
- Life Sciences
- Materials
- Tech Scout
- Unparalleled Data Quality
- Higher Quality Content
- 60% Fewer Hallucinations
Browse by: Latest US Patents, China's latest patents, Technical Efficacy Thesaurus, Application Domain, Technology Topic, Popular Technical Reports.
© 2025 PatSnap. All rights reserved.Legal|Privacy policy|Modern Slavery Act Transparency Statement|Sitemap|About US| Contact US: help@patsnap.com