Passenger detecting method based on Haar-PCA characteristic and probability neural network
A probabilistic neural network and pedestrian detection technology, applied in the field of image processing, can solve the problems of reducing the detection rate and lengthy BPANN training time, and achieve the effects of reducing dimensionality, improving classification performance, and reducing training time
- Summary
- Abstract
- Description
- Claims
- Application Information
AI Technical Summary
Problems solved by technology
Method used
Image
Examples
Embodiment Construction
[0035] The following will clearly and completely describe the technical solutions in the embodiments of the present invention with reference to the drawings in the embodiments of the present invention.
[0036] Step 1: Manually select a large number of pictures of pedestrians and non-pedestrians, and mark them as positive and negative samples respectively. All positive and negative samples are normalized to a dimension of 32 pixels in length and width. Let the total number of positive and negative samples be n.
[0037] Step 2: Take any positive and negative sample S i Characterize with Haar features to generate Haar feature vector H i , (i=1, 2, . . . , n).
[0038] Step 2-1: There are various types of Haar rectangle features. Considering that pedestrians present more horizontal and vertical line features in the image, select such as figure 2 The 10 classes of Haar features shown. There are white and black rectangles in the Haar feature template, and the size of a certa...
PUM
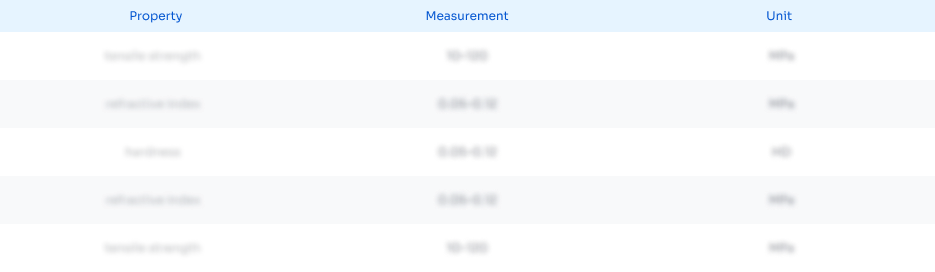
Abstract
Description
Claims
Application Information
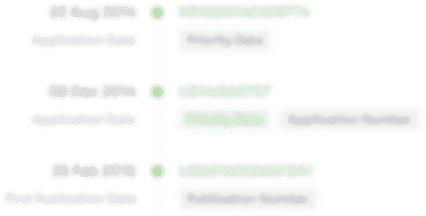
- R&D Engineer
- R&D Manager
- IP Professional
- Industry Leading Data Capabilities
- Powerful AI technology
- Patent DNA Extraction
Browse by: Latest US Patents, China's latest patents, Technical Efficacy Thesaurus, Application Domain, Technology Topic, Popular Technical Reports.
© 2024 PatSnap. All rights reserved.Legal|Privacy policy|Modern Slavery Act Transparency Statement|Sitemap|About US| Contact US: help@patsnap.com