Grating-scale-measuring-error dynamic compensation method based on deep learning
A technology of measurement error and dynamic compensation, which is applied in measurement devices, complex mathematical operations, and optical devices, etc., can solve problems such as the application limitation of the grating ruler, the destruction of signal structure characteristics, and the difficulty in effectively improving the measurement accuracy of the grating ruler.
- Summary
- Abstract
- Description
- Claims
- Application Information
AI Technical Summary
Problems solved by technology
Method used
Image
Examples
Embodiment Construction
[0059] refer to figure 1 , the present invention provides a method for dynamic compensation of grating ruler measurement error based on deep learning, comprising steps:
[0060] S1. Obtain the error data after collecting the measurement data of the grating ruler and the laser interferometer, and simultaneously use multiple sensors to measure and obtain the action intensity values of various interference factors corresponding to the error data;
[0061] S2. Based on the empirical mode decomposition algorithm, the error data is decomposed into multiple IMF components, and the Hilbert edge spectrum of each IMF component is obtained by solving;
[0062] S3. The action intensity values of various interference factors corresponding to the error data and the Hilbert edge spectrum of multiple IMF components are used as input data, and after the trained CNN neural network is used for identification and calculation, the corresponding output label function is obtained. ;
[0063] S...
PUM
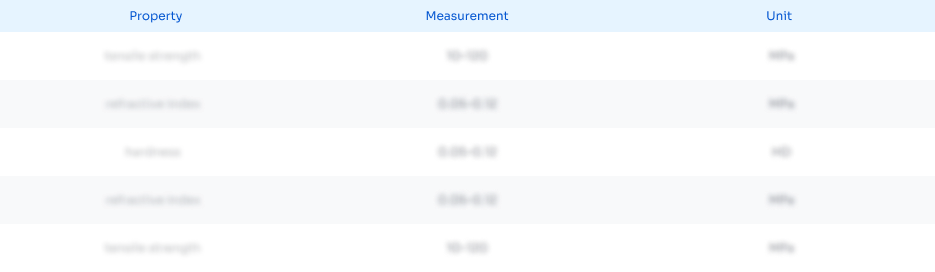
Abstract
Description
Claims
Application Information
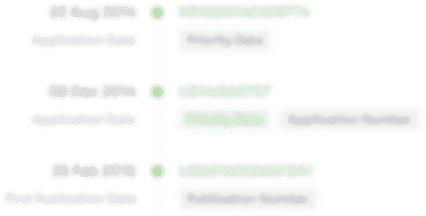
- R&D
- Intellectual Property
- Life Sciences
- Materials
- Tech Scout
- Unparalleled Data Quality
- Higher Quality Content
- 60% Fewer Hallucinations
Browse by: Latest US Patents, China's latest patents, Technical Efficacy Thesaurus, Application Domain, Technology Topic, Popular Technical Reports.
© 2025 PatSnap. All rights reserved.Legal|Privacy policy|Modern Slavery Act Transparency Statement|Sitemap|About US| Contact US: help@patsnap.com