Wind power short-term interval prediction method based on RT reconstructed EEMD-RVM combined model
A technology that combines models and forecasting methods, applied in forecasting, instrumentation, data processing applications, etc., and can solve problems such as many parameters, only point forecasting, and large amount of calculation.
- Summary
- Abstract
- Description
- Claims
- Application Information
AI Technical Summary
Problems solved by technology
Method used
Image
Examples
Embodiment
[0117] Considering the random fluctuation of wind power, the present invention proposes a short-term interval prediction method of wind power based on the reconstructed EEMD-RVM combination model based on the run length detection method. On the one hand, the ensemble empirical mode decomposition is introduced to decompose the original wind power sequence to obtain multiple IMF components and RES components, which reduces the complexity of the data, and the run length detection method is introduced to detect the fluctuation degree of each IMF component and RES component. Components with similar fluctuations and changes are reconstructed into three types of new components with typical characteristics: random components, detail components and trend components in the order of fine-to-coarse, which further reduces the scale of the forecasting model and shortens the running time; on the other hand For each new component, a correlation vector machine is used to establish an interval p...
PUM
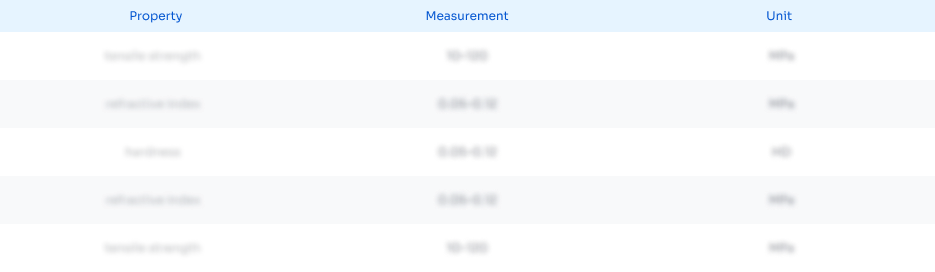
Abstract
Description
Claims
Application Information
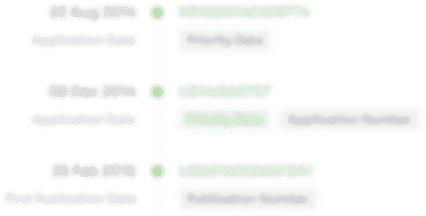
- R&D Engineer
- R&D Manager
- IP Professional
- Industry Leading Data Capabilities
- Powerful AI technology
- Patent DNA Extraction
Browse by: Latest US Patents, China's latest patents, Technical Efficacy Thesaurus, Application Domain, Technology Topic, Popular Technical Reports.
© 2024 PatSnap. All rights reserved.Legal|Privacy policy|Modern Slavery Act Transparency Statement|Sitemap|About US| Contact US: help@patsnap.com