Method for checking class attendance on basis of multi-face data acquisition strategies and deep learning
A technology of data collection and deep learning, applied in character and pattern recognition, recording/indicating event time, instruments, etc., can solve problems such as difficulty in unified collection, time-consuming and labor-intensive face data collection, poor recognition rate, etc., to achieve The effect of improving the face recognition rate
- Summary
- Abstract
- Description
- Claims
- Application Information
AI Technical Summary
Problems solved by technology
Method used
Image
Examples
Embodiment approach
[0083] The invention combines the AdaBoost algorithm with the skin color model, locates the face position through the AdaBoost algorithm, and then uses the skin color model to perform skin color verification, which can greatly reduce the false detection rate during face detection. The main implementation methods are as follows:
[0084] ①Using the Adaboost algorithm to generate a classifier for face detection, and perform preliminary face detection.
[0085] ②Use the skin color model to verify the face area initially determined by Adaboost, and distinguish the skin color area and the non-skin color area in the image by comparing the pixels in the image with the "standard skin color". When setting the "standard skin tone" range, three color spaces are used: RGB color space, HSV color space, and YCbCr color space.
[0086] Set two RGB standard skin tone models. Threshold range of model one: G>40, B>20, R>G, R>B, MAX(R,G,B)-MIN(R,G,B)>15; threshold range of model two: R> 220, ...
PUM
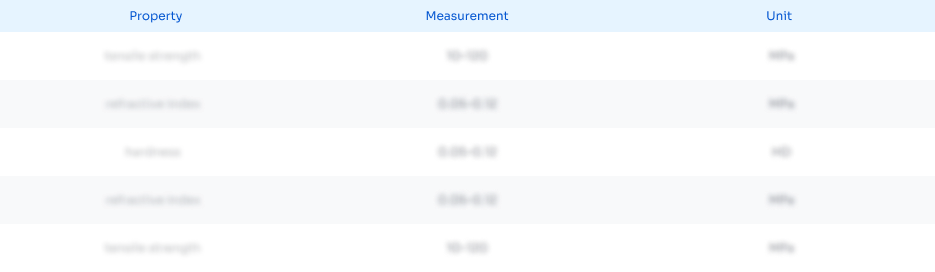
Abstract
Description
Claims
Application Information
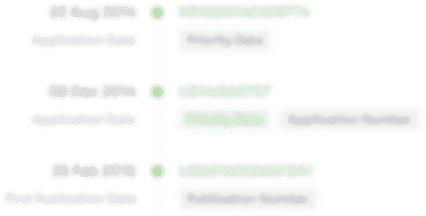
- R&D Engineer
- R&D Manager
- IP Professional
- Industry Leading Data Capabilities
- Powerful AI technology
- Patent DNA Extraction
Browse by: Latest US Patents, China's latest patents, Technical Efficacy Thesaurus, Application Domain, Technology Topic, Popular Technical Reports.
© 2024 PatSnap. All rights reserved.Legal|Privacy policy|Modern Slavery Act Transparency Statement|Sitemap|About US| Contact US: help@patsnap.com