Electrocardiography comprehensive sorting method based on deep learning algorithm
A technology of deep learning and classification methods, applied in medical science, sensors, diagnostic recording/measurement, etc., can solve problems such as easy misclassification, low clinical reliability and accuracy, and failure to meet classification needs, so as to improve accuracy , the effect of improving the accuracy rate
- Summary
- Abstract
- Description
- Claims
- Application Information
AI Technical Summary
Problems solved by technology
Method used
Image
Examples
Embodiment 1
[0050] A comprehensive classification method of electrocardiogram based on deep learning algorithm, its flow chart is as follows figure 1 As shown, it specifically includes the following steps:
[0051] a. (1) Obtain single-lead ECG waveform data and ECG additional information, and intercept the data with a length of 10 seconds as the original ECG waveform data according to the single-lead ECG waveform data, in which the single-lead ECG waveform data and ECG additional information Information can be obtained through physical examination results, existing databases such as the ECG waveform database (CSE), or other means. Additional information on the ECG includes gender, height, bust, weight, fat percentage, and race .
[0052] (2) as required, the original electrocardiogram waveform data obtained in step (1) can be denoised, and the denoised process includes the following steps:
[0053] i1. Use a high-pass filter to remove baseline drift noise;
[0054] i2. Confirm whether...
Embodiment 2
[0074] A comprehensive classification method of electrocardiogram based on deep learning algorithm, its flow chart is as follows figure 1 As shown, it specifically includes the following steps:
[0075] a. (1) Obtain single-lead ECG waveform data and ECG additional information, and intercept the data with a length of 8 seconds as the original ECG waveform data according to the single-lead ECG waveform data, in which the single-lead ECG waveform data and ECG additional information Information can be obtained through physical examination results, existing databases such as the ECG waveform database (CSE), or other means. Additional information on the ECG includes gender, height, bust, weight, fat percentage, and race .
[0076] (2) as required, the original electrocardiogram waveform data obtained in step (1) can be denoised, and the denoised process includes the following steps:
[0077] i1. Use a high-pass filter to remove baseline drift noise;
[0078] i2. Confirm whether ...
Embodiment 3
[0098] A comprehensive classification method of electrocardiogram based on deep learning algorithm, its flow chart is as follows figure 1 As shown, it specifically includes the following steps:
[0099] a. (1) Obtain single-lead ECG waveform data and ECG additional information, and intercept the data with a length of 16 seconds as the original ECG waveform data according to the single-lead ECG waveform data, in which the single-lead ECG waveform data and ECG additional information Information can be obtained from physical examination results, existing databases such as the ECG waveform database (CSE), or other means. Additional information on the ECG includes gender, height, bust, weight, fat percentage, and race .
[0100] (2) as required, the original electrocardiogram waveform data obtained in step (1) can be denoised, and the denoised process includes the following steps:
[0101] i1. Use a high-pass filter to remove baseline drift noise;
[0102] i2. Confirm whether th...
PUM
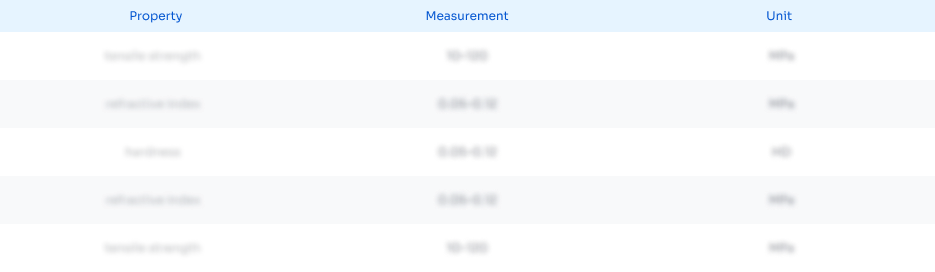
Abstract
Description
Claims
Application Information
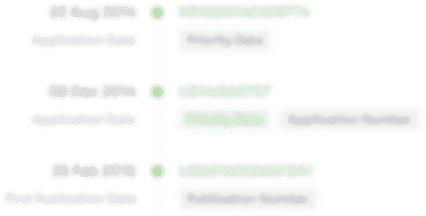
- R&D Engineer
- R&D Manager
- IP Professional
- Industry Leading Data Capabilities
- Powerful AI technology
- Patent DNA Extraction
Browse by: Latest US Patents, China's latest patents, Technical Efficacy Thesaurus, Application Domain, Technology Topic, Popular Technical Reports.
© 2024 PatSnap. All rights reserved.Legal|Privacy policy|Modern Slavery Act Transparency Statement|Sitemap|About US| Contact US: help@patsnap.com