Homogenous data set characteristic quality visualization method
A data set and data subset technology, applied in the field of machine learning, can solve the problem of no quantitative research or visual analysis of feature stability and feature correlation, and achieve the effect of helping manual feature selection, increasing intuitive understanding and strong interpretability
- Summary
- Abstract
- Description
- Claims
- Application Information
AI Technical Summary
Problems solved by technology
Method used
Image
Examples
Embodiment 1
[0043] Table 1
[0044]
[0045] This embodiment is a conversion rate model of an advertiser, and the isomorphic data set D is the sample data of the customer, and the label is whether to convert or not. In this example, the steps of HoDFQV, a feature quality visualization method for isomorphic datasets, are as follows:
[0046] Step 1, given the isomorphic data set D, D is the conversion rate sample set, given the feature f, f is dayofweek, that is, the day of the week, and constructs a feature category value set V = {1, 2, 3, 4, 5, 6, 7}, respectively represent Monday to Sunday, divide the isomorphic data set D into K=3 data subsets according to the week, that is, D={1, 2, 3}.
[0047] Step 2, for each data subset d in the isomorphic data set D, calculate its overall positive sample incidence rate r(d), the calculation formula is r(d)=pos(d) / ins(d), where pos (d), ins(d) represent the number of positive samples and the total number of samples in d; when d=1, r(1)=767 / 84...
Embodiment 2
[0057] This example is an advertising conversion rate model, where the data set is sample data of a customer in an e-commerce industry, and the label is whether to convert or not. In this example, the steps of HoDFEP, a visual evaluation process of homogeneous dataset features, are as follows:
[0058] Step 1. Given a homogeneous data set D and a feature set F, the number of features to be selected is N. The feature set F includes two features {hourofday, dayofweek} respectively representing the time of day and the day of the week, and the number of features to be selected is N=1.
[0059] Step 2, calculate the index data of the feature hourofday and dayofweek respectively, including the number of category values, incidence rate, normalized incidence rate, drift degree, comprehensive incidence rate, etc., to form an index set M; draw the feature quality map of the feature hourofday and dayofweek, Constitute a graph set G.
[0060] Step 3, according to the index set M and the...
PUM
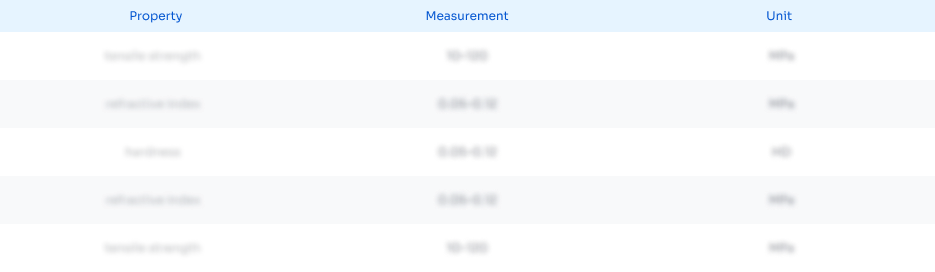
Abstract
Description
Claims
Application Information
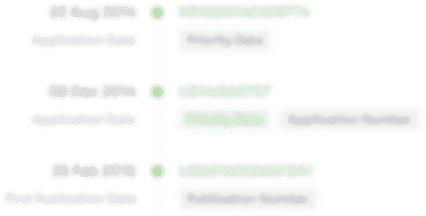
- R&D
- Intellectual Property
- Life Sciences
- Materials
- Tech Scout
- Unparalleled Data Quality
- Higher Quality Content
- 60% Fewer Hallucinations
Browse by: Latest US Patents, China's latest patents, Technical Efficacy Thesaurus, Application Domain, Technology Topic, Popular Technical Reports.
© 2025 PatSnap. All rights reserved.Legal|Privacy policy|Modern Slavery Act Transparency Statement|Sitemap|About US| Contact US: help@patsnap.com