SAR image target detection method based on convolutional neural network
A convolutional neural network and target detection technology, applied in neural learning methods, biological neural network models, neural architectures, etc., can solve the problem that SAR image data cannot meet the large amount of training data of convolutional neural network models, and improve the detection accuracy. rate effect
- Summary
- Abstract
- Description
- Claims
- Application Information
AI Technical Summary
Problems solved by technology
Method used
Image
Examples
Embodiment Construction
[0038] refer to figure 1 , the SAR image target detection method of the present invention mainly has three stages of model design, training and testing, and concrete steps are as follows:
[0039] 1. Model design stage
[0040] Step 1, design the convolutional neural network model M 0 '.
[0041] refer to figure 2 , convolutional neural network M' 0 It consists of two parts: feature extraction and classification judgment, among which:
[0042] The feature extraction part includes three convolutional layers, the convolutional layer is followed by a nonlinear layer, and the first two nonlinear layers are followed by a maximum pooling layer;
[0043] The classification decision part includes two fully connected layers, the first fully connected layer is followed by a nonlinear layer, the second fully connected layer is followed by a flexible maximum layer and a classification loss function layer, which is expressed as:
[0044] m 0 '=[I,C 1 ,R,Pm,C 2 ,R,Pm,C 3 ,R,Fc 1 ,...
PUM
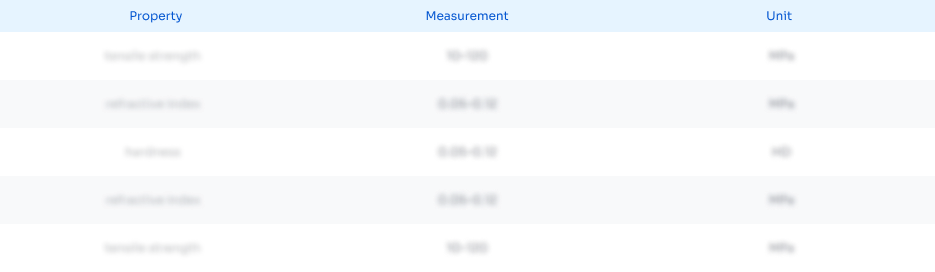
Abstract
Description
Claims
Application Information
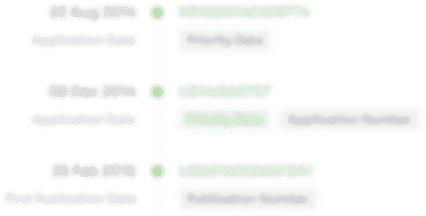
- R&D
- Intellectual Property
- Life Sciences
- Materials
- Tech Scout
- Unparalleled Data Quality
- Higher Quality Content
- 60% Fewer Hallucinations
Browse by: Latest US Patents, China's latest patents, Technical Efficacy Thesaurus, Application Domain, Technology Topic, Popular Technical Reports.
© 2025 PatSnap. All rights reserved.Legal|Privacy policy|Modern Slavery Act Transparency Statement|Sitemap|About US| Contact US: help@patsnap.com