Random convolutional neural network-based high-resolution image scene classification method
A high-resolution image and neural network technology, applied to computer components, instruments, calculations, etc.
- Summary
- Abstract
- Description
- Claims
- Application Information
AI Technical Summary
Problems solved by technology
Method used
Image
Examples
Embodiment Construction
[0039] The present invention will be further described below in conjunction with the accompanying drawings and specific embodiments.
[0040] The present invention uses the deep learning method to perform hierarchical feature learning on the scene classification problem, combines the aggregation model to further improve the generalization ability of the model, and uses the gradient lifting method to perform model aggregation, so that the feature learning result is robust.
[0041] Introduce a deep convolutional network, and use a deep convolutional neural network for hierarchical feature learning of scene images. Deep convolutional network is a method to simulate the hierarchical feature recognition of human brain. Image features are often learned layer by layer from low level to high level, so the optimal feature expression can be guaranteed to be learned. The reason for learning features in this way is that for human brain vision, scene recognition is often performed hierar...
PUM
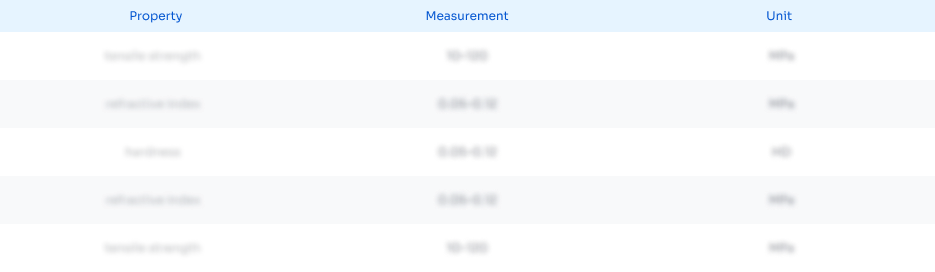
Abstract
Description
Claims
Application Information
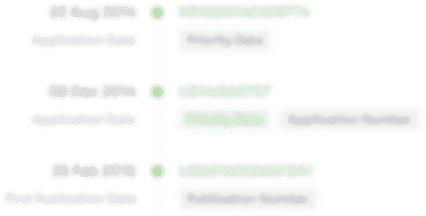
- R&D Engineer
- R&D Manager
- IP Professional
- Industry Leading Data Capabilities
- Powerful AI technology
- Patent DNA Extraction
Browse by: Latest US Patents, China's latest patents, Technical Efficacy Thesaurus, Application Domain, Technology Topic, Popular Technical Reports.
© 2024 PatSnap. All rights reserved.Legal|Privacy policy|Modern Slavery Act Transparency Statement|Sitemap|About US| Contact US: help@patsnap.com