Improved-Fisher-based chemical process fault diagnosis method
A chemical process and fault diagnosis technology, applied in the chemical process fault diagnosis, based on the improved nuclear Fisher's chemical process fault diagnosis field, can solve the problems of fuzzy, aliased data classification, etc., to improve the distribution, facilitate the classification, improve the classification The effect of accuracy
- Summary
- Abstract
- Description
- Claims
- Application Information
AI Technical Summary
Problems solved by technology
Method used
Image
Examples
Embodiment 1
[0034] A chemical process fault diagnosis method based on improved Kernel Fisher proposed by the present invention is based on the Kernel Fisher method, and is improved from the two aspects of inter-class dispersion and boundary data classification method, which improves the accuracy of fault diagnosis . figure 1 It is a chemical process fault diagnosis method based on the improved Kernel Fisher of the present invention, which also includes a flow chart of corresponding steps in this embodiment.
[0035] From figure 1 It can be seen that the present invention comprises the following steps:
[0036] Step A, input raw data;
[0037] Step B, normalize processing, and output training set and test set;
[0038] Step C, for different types of data output in step B, perform the following operations respectively:
[0039] C.1 Use the cross-validation method to select the kernel parameters for the data in the training set, and obtain the kernel Fisher method for improving the class...
Embodiment 2
[0073] In order to test the effectiveness of the fault diagnosis method proposed by the present invention and the detailed steps in Embodiment 1, this embodiment uses the Tennessee-Eastman (TE) process to verify the correctness of the present invention and Embodiment 1. As the data source of various statistical data analysis algorithms, TE process has been widely recognized and used. The TE process includes five main process units: reactor, condenser, compressor, separator, and stripper, as well as 12 controlled variables, 41 observed variables, and 20 types of typical faults. For a detailed introduction to the TE process, see the literature: In 2010, Wang Ting, titled "Research on Real-time Optimization Technology for TE Process".
[0074] In this embodiment, faults 4, 9, and 11 are selected for relevant experimental verification, and characteristic variables {51, 9} among 53 characteristic variables are selected to form each fault sample. For each type of fault type, two se...
Embodiment 3
[0083] TE process fault diagnosis, a total of 21 types of faults, these 21 types of faults are pre-set, of which the first 7 types of faults are all steps, and the 3rd, 4th, 5th, and 7th types of faults are selected as the research objects . For each fault type, two sets of data are taken, namely training data and test data, each set of data has 100 samples, and each sample is composed of all 52 feature variables. The training set and test set each contain 400 samples.
[0084] The kernel Fisher projection is used for the training set samples, and the obtained projection images on the first and second characteristic axes are as follows: Figure 4 As shown, the kernel parameter δ is set to 200. Figure 4 , the abscissa is the first characteristic axis, and the ordinate is the second characteristic axis; the circle represents fault 3, the five-pointed star represents fault 4, the triangle represents fault 5, and the square represents fault 7. In the same way, the kernel Fishe...
PUM
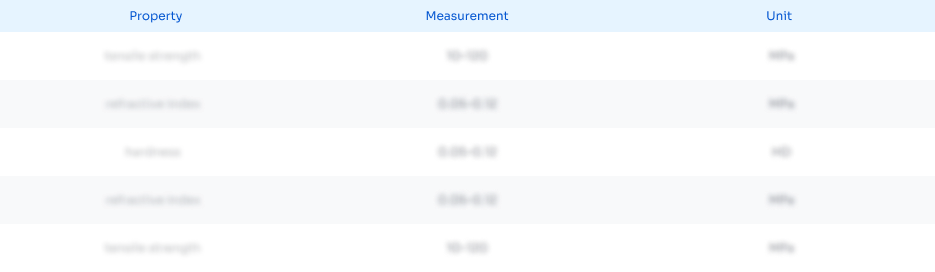
Abstract
Description
Claims
Application Information
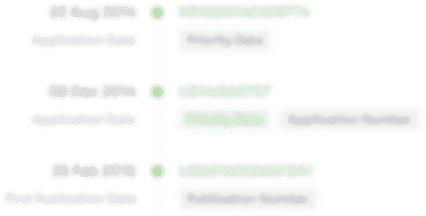
- R&D
- Intellectual Property
- Life Sciences
- Materials
- Tech Scout
- Unparalleled Data Quality
- Higher Quality Content
- 60% Fewer Hallucinations
Browse by: Latest US Patents, China's latest patents, Technical Efficacy Thesaurus, Application Domain, Technology Topic, Popular Technical Reports.
© 2025 PatSnap. All rights reserved.Legal|Privacy policy|Modern Slavery Act Transparency Statement|Sitemap|About US| Contact US: help@patsnap.com