A Method of Fault Classification and Diagnosis Based on Nonlinear Similarity Index
A technology of similarity index and diagnosis method, which is applied in the field of fault classification and diagnosis based on nonlinear similarity index, can solve the problems of insufficient number of samples and reduced restriction of model establishment, and achieve suppression of negative interference, reduction of error matching rate, The effect of limiting force reduction
- Summary
- Abstract
- Description
- Claims
- Application Information
AI Technical Summary
Problems solved by technology
Method used
Image
Examples
Embodiment Construction
[0024] The present invention will be described in detail below in conjunction with the accompanying drawings and specific embodiments.
[0025] like figure 1 As shown, the present invention discloses a fault classification and diagnosis method based on nonlinear similarity index, and the specific implementation steps of the method are as follows:
[0026] Step 1: Collect sampling data under normal operating conditions of the production process to form a data matrix X 0 ∈ R n×m , collect the sampling data of the production process under different fault operation states to form different reference fault data sets Among them, n is the number of training samples, m is the number of process measurement variables, subscript c=1, 2, ..., C represents the c-th reference fault type, N c is the number of samples available for the c-th fault, R is a set of real numbers, R n×m Represents an n×m-dimensional real number matrix.
[0027] Step 2: For matrix X 0 Perform standardization ...
PUM
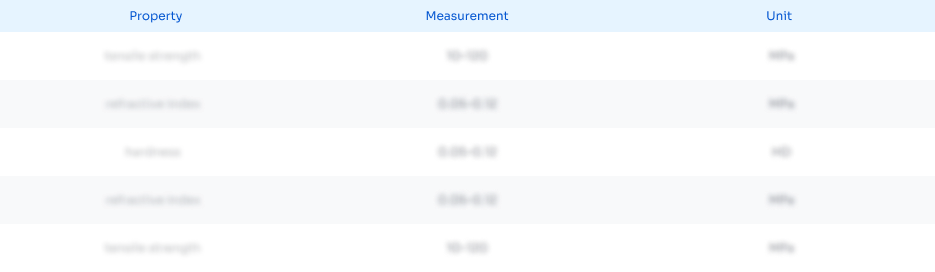
Abstract
Description
Claims
Application Information
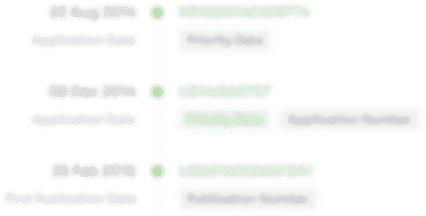
- R&D Engineer
- R&D Manager
- IP Professional
- Industry Leading Data Capabilities
- Powerful AI technology
- Patent DNA Extraction
Browse by: Latest US Patents, China's latest patents, Technical Efficacy Thesaurus, Application Domain, Technology Topic, Popular Technical Reports.
© 2024 PatSnap. All rights reserved.Legal|Privacy policy|Modern Slavery Act Transparency Statement|Sitemap|About US| Contact US: help@patsnap.com