Deep convolution neural network-based traffic flow density estimation method
A convolutional neural network, traffic density technology, applied in biological neural network models, neural architectures, counting of randomly distributed items, etc., can solve the problems of inability to obtain the number of vehicles, lack of vehicle density detection accuracy and quantitative analysis, etc. Achieve the effect of avoiding background modeling difficulties, easy learning, and avoiding inaccurate features
- Summary
- Abstract
- Description
- Claims
- Application Information
AI Technical Summary
Problems solved by technology
Method used
Image
Examples
Embodiment Construction
[0048] Specific embodiments of the present invention will be described below in conjunction with the accompanying drawings, so that those skilled in the art can better understand the present invention.
[0049] The method for estimating traffic density and counting vehicles based on a deep convolutional neural network, based on a large number of samples of different traffic densities, uses a convolutional neural network to automatically extract feature maps with a high degree of discrimination, and multi-scale pyramidal image blocks input multiple The scale convolutional neural network forms an image density and vehicle counting model. By selecting the region of interest, the instantaneous traffic density of a fixed-length interval can be calculated, which greatly improves the accuracy and real-time performance of traffic density detection. In the training phase, train multiple convolutional neural networks with input images of different scales, learn the essential characterist...
PUM
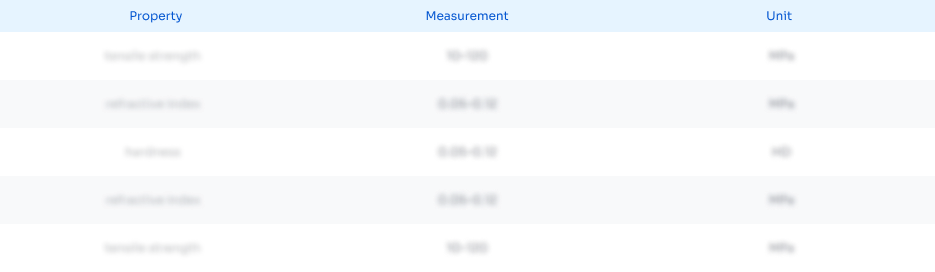
Abstract
Description
Claims
Application Information
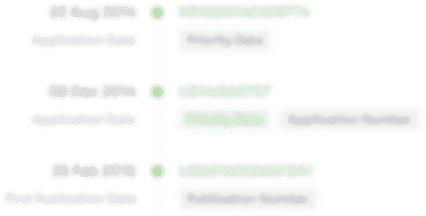
- R&D Engineer
- R&D Manager
- IP Professional
- Industry Leading Data Capabilities
- Powerful AI technology
- Patent DNA Extraction
Browse by: Latest US Patents, China's latest patents, Technical Efficacy Thesaurus, Application Domain, Technology Topic, Popular Technical Reports.
© 2024 PatSnap. All rights reserved.Legal|Privacy policy|Modern Slavery Act Transparency Statement|Sitemap|About US| Contact US: help@patsnap.com