SVM (support vector machine) parameter selection method based on multi-kernel function adaptive fusion
A support vector machine and parameter selection technology, which is applied to computer parts, instruments, character and pattern recognition, etc., can solve the problem of ideal classification effect of support vector machine
- Summary
- Abstract
- Description
- Claims
- Application Information
AI Technical Summary
Problems solved by technology
Method used
Image
Examples
Embodiment Construction
[0036] The present invention will be further described below in conjunction with accompanying drawing:
[0037] Problem Description:
[0038] The structure diagram of the support vector regression machine is as follows: figure 1 As shown, the key of support vector machine is the introduction of kernel function. The kernel function cleverly solves the problem of dimensionality disaster caused by mapping low-dimensional vectors to high-dimensional ones, and improves the nonlinear processing ability of machine learning. Each kernel function has its own characteristics, and support vector regression machines based on different kernel functions have different generalization capabilities. Kernel function is one of the most important problems in support vector machine, how to choose the appropriate kernel function is also a difficult problem without theoretical basis in support vector machine.
[0039] Let K(x i ,x j ) represents the kernel function, where x i , x j for the sa...
PUM
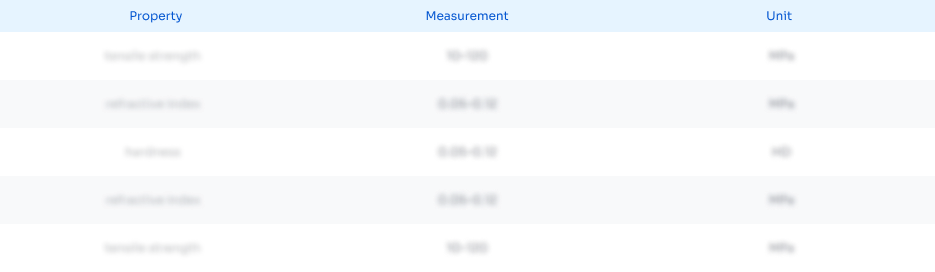
Abstract
Description
Claims
Application Information
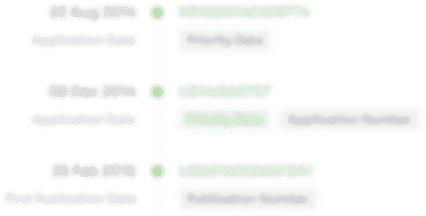
- R&D
- Intellectual Property
- Life Sciences
- Materials
- Tech Scout
- Unparalleled Data Quality
- Higher Quality Content
- 60% Fewer Hallucinations
Browse by: Latest US Patents, China's latest patents, Technical Efficacy Thesaurus, Application Domain, Technology Topic, Popular Technical Reports.
© 2025 PatSnap. All rights reserved.Legal|Privacy policy|Modern Slavery Act Transparency Statement|Sitemap|About US| Contact US: help@patsnap.com