Method for classifying grid equipment based on convolution neural network
A convolutional neural network and power grid equipment technology, which is applied to biological neural network models, instruments, character and pattern recognition, etc., can solve problems such as impracticality and inaccurate test data classification results, and achieve reduction in size and increase in size. Effects of size and accuracy improvement
- Summary
- Abstract
- Description
- Claims
- Application Information
AI Technical Summary
Problems solved by technology
Method used
Image
Examples
Embodiment Construction
[0024] The present invention will be further described below in conjunction with the accompanying drawings. The following examples are only used to illustrate the technical solution of the present invention more clearly, but not to limit the protection scope of the present invention.
[0025] A method for classifying power grid equipment based on convolutional neural networks, comprising the following steps:
[0026] Step 1, construct training set and test set.
[0027] By taking photos on the spot, collect images of six major power grid equipment, 24 images for each category, and divide them into training images and test images in a ratio of 3:1.
[0028] Step 2. Construct the grid equipment classification label document corresponding to the grid equipment image.
[0029] Each grid equipment image corresponds to a grid equipment classification label file, which stores the reading path and file name of the corresponding grid equipment image, where file name = name + number l...
PUM
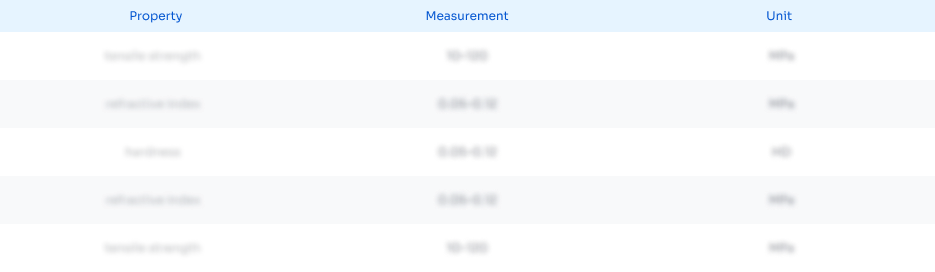
Abstract
Description
Claims
Application Information
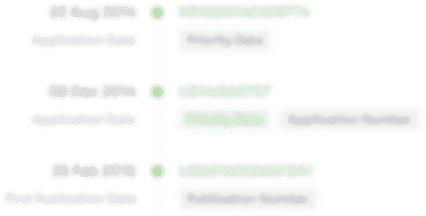
- R&D Engineer
- R&D Manager
- IP Professional
- Industry Leading Data Capabilities
- Powerful AI technology
- Patent DNA Extraction
Browse by: Latest US Patents, China's latest patents, Technical Efficacy Thesaurus, Application Domain, Technology Topic, Popular Technical Reports.
© 2024 PatSnap. All rights reserved.Legal|Privacy policy|Modern Slavery Act Transparency Statement|Sitemap|About US| Contact US: help@patsnap.com