Deep belief network and relevance vector machine fusion-based lithium battery residual life prediction method
A correlation vector machine, deep confidence technology, applied in the field of lithium-ion battery cycle life prediction, which can solve problems such as dependence, expensive investment, and limited fault prediction performance
- Summary
- Abstract
- Description
- Claims
- Application Information
AI Technical Summary
Problems solved by technology
Method used
Image
Examples
specific Embodiment approach 1
[0026] Specific implementation mode one: combine Figure 1 to Figure 8 Specifically illustrate this embodiment, the method for predicting the remaining life of a lithium battery based on the fusion of a deep belief network and a correlation vector machine described in this embodiment, the method includes the following steps:
[0027] Step 1. Obtain the lithium battery capacity degradation data set based on the charge and discharge cycle, that is, the original data set; preprocess the data, that is, normalize the data to the interval [0,1], and divide the data set into two The data sets are respectively a training data set and a test data set; the data before the starting point of prediction (SP) are used for training, which is the training data, and the data after the SP are used for testing, which is the test data;
[0028] Step 2, building a fusion model of DBN and RVM, that is, building a deep belief network DBN model and a correlation vector machine RVM model;
[0029] St...
PUM
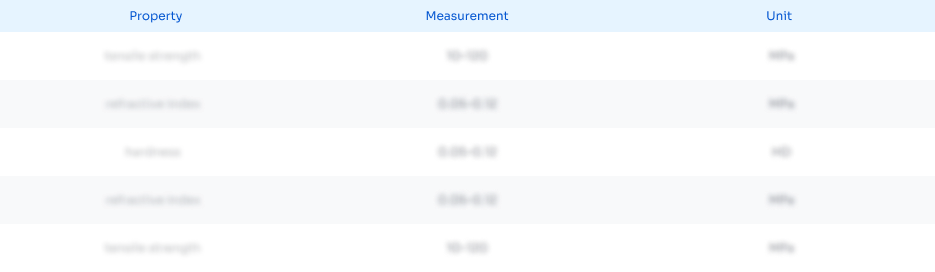
Abstract
Description
Claims
Application Information
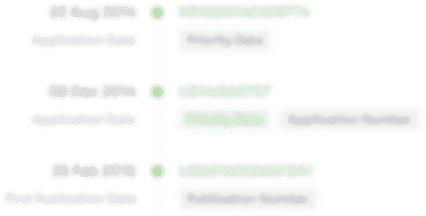
- R&D
- Intellectual Property
- Life Sciences
- Materials
- Tech Scout
- Unparalleled Data Quality
- Higher Quality Content
- 60% Fewer Hallucinations
Browse by: Latest US Patents, China's latest patents, Technical Efficacy Thesaurus, Application Domain, Technology Topic, Popular Technical Reports.
© 2025 PatSnap. All rights reserved.Legal|Privacy policy|Modern Slavery Act Transparency Statement|Sitemap|About US| Contact US: help@patsnap.com