A traffic signal adaptive control method based on deep reinforcement learning
An adaptive control, traffic signal technology, applied in the direction of traffic signal control, neural learning method, biological neural network model, etc., can solve the problems of inaccurate signal control strategy formulation, incomplete traffic state perception, etc., to solve the problem of traffic state perception Inaccurate, to achieve the effect of accurate perception
- Summary
- Abstract
- Description
- Claims
- Application Information
AI Technical Summary
Problems solved by technology
Method used
Image
Examples
Embodiment Construction
[0034] The present invention will be further described below in conjunction with accompanying drawing.
[0035] Such as figure 1 As shown, a traffic signal adaptive control method based on deep reinforcement learning, including the following steps:
[0036] Step 1. Define traffic signal control Agent, state space S, action space A and reward function r, specifically including the following sub-steps:
[0037] Step 1.1, the traffic signal control agent uses the deep reinforcement learning method to build a deep neural network Q V For the value network, the initialization experience playback memory pool D is empty, and the neural network of the present invention adopts a convolutional neural network, followed by an input layer, 3 convolutional layers, 1 fully connected layer and 4 output layers, and the input layer is the current The traffic state s, the output layer estimates Q for the value of all actions in the current traffic state V (s, a); experience playback memory poo...
PUM
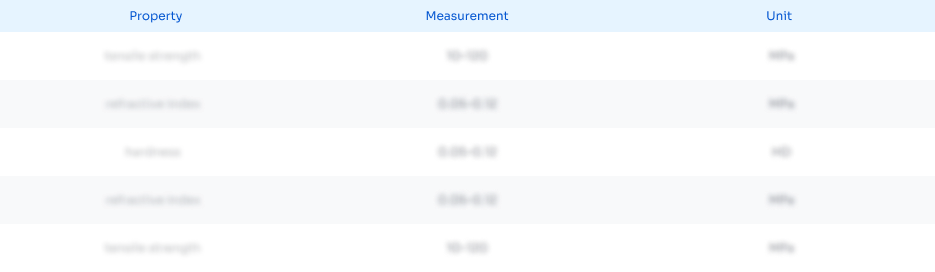
Abstract
Description
Claims
Application Information
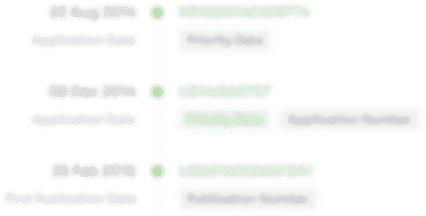
- R&D
- Intellectual Property
- Life Sciences
- Materials
- Tech Scout
- Unparalleled Data Quality
- Higher Quality Content
- 60% Fewer Hallucinations
Browse by: Latest US Patents, China's latest patents, Technical Efficacy Thesaurus, Application Domain, Technology Topic, Popular Technical Reports.
© 2025 PatSnap. All rights reserved.Legal|Privacy policy|Modern Slavery Act Transparency Statement|Sitemap|About US| Contact US: help@patsnap.com